
LAO GRUESO Oscar
Recommendations: 0
Review: 1
Review: 1
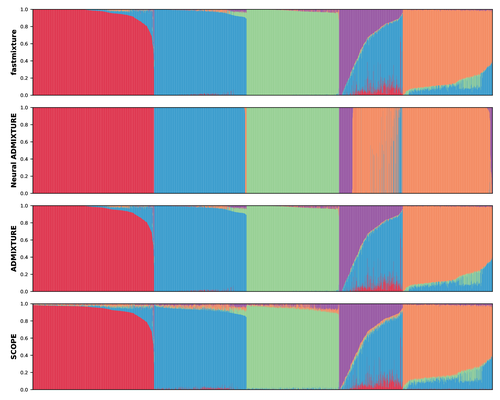
Faster model-based estimation of ancestry proportions
fastmixture generates fast and accurate estimates of global ancestry proportions and ancestral allele frequencies
Recommended by Matteo Fumagalli based on reviews by Oscar Lao Grueso and 2 anonymous reviewersThe estimation of ancestry proportions in individuals is an important analysis in both evolutionary biology and medical genetics. However, popular tools like ADMIXTURE (Alexander et al. 2009) and STRUCTURE (Pritchard et al. 2000) do not scale well with the large amount of data currently available. Recent alternative methods, such as SCOPE (Chiu et al. 2022), favour scalability over accuracy.
In this study, Santander and coworkers introduce a new software, called fastmixture, which estimates ancestry proportions and ancestral allele frequencies using novel implementations for initialisation and convergence of its model-based algorithm (Santander et al. 2024). In simulated datasets, fastmixture displays desirable properties of speed and accuracy, with its performance surpassing commonly used software (Alexander et al. 2009, Pritchard et al. 2000, Chiu et al. 2022, Mantes et al. 2023). fastmixture is almost 30 times faster than ADMIXTURE under a complex model with five ancestral populations, while retaining similar accuracy levels. When applied to data from the 1000 Genomes Project (1000 Genomes Project Consortium 2025), fastmixture recapitulated expected levels of global ancestry. The new software is freely available on GitHub with an accessible documentation. fastmixture accepts input files in PLINK format.
It remains an open question whether extensive parameter tuning could increase the scalability and accuracy of established methods. A comprehensive assessment of fastmixture over a wide range of data processing options (Hemstrom et al. 2024) is also missing. Finally, whether model-based approaches are fully scalable to ever increasing biobank datasets is still under debate. Nevertheless, the superior computational performance of fastmixture is evident and it is likely that this new software will soon replace existing popular tools to estimate global ancestry proportions.
References
Alexander DH, Novembre J, Lange K. Fast model-based estimation of ancestry in unrelated individuals. Genome Res. 2009;19(9):1655-1664. https://doi.org/10.1101/gr.094052.109
Pritchard JK, Stephens M, Donnelly P. Inference of population structure using multilocus genotype data. Genetics. 2000;155(2):945-959. https://doi.org/10.1093/genetics/155.2.945
Chiu AM, Molloy EK, Tan Z, Talwalkar A, Sankararaman S. Inferring population structure in biobank-scale genomic data. Am J Hum Genet. 2022;109(4):727-737. https://doi.org/10.1016/j.ajhg.2022.02.015
Santander CG, Refoyo Martinez A, Meisner J. Faster model-based estimation of ancestry proportions. bioRxiv 2024; ver.2 peer-reviewed and recommended by PCI Evol Biol. https://doi.org/10.1101/2024.07.08.602454
Mantes AD, Montserrat DM, Bustamante CD, Giró-I-Nieto X, Ioannidis AG. Neural ADMIXTURE for rapid genomic clustering. Nat Comput Sci. 2023;3(7):621-629. https://doi.org/10.1038/s43588-023-00482-7
1000 Genomes Project Consortium, Auton A, Brooks LD, et al. A global reference for human genetic variation. Nature. 2015;526(7571):68-74. https://doi.org/10.1038/nature15393
Hemstrom W, Grummer JA, Luikart G, Christie MR. Next-generation data filtering in the genomics era. Nat Rev Genet. 2024;25(11):750-767. https://doi.org/10.1038/s41576-024-00738-6