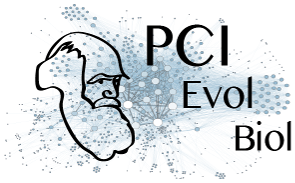
More intense symptoms, more treatment, more drug-resistance: coevolution of virulence and drug-resistance
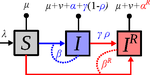
Treating symptomatic infections and the co-evolution of virulence and drug resistance
Abstract
Recommendation: posted 04 November 2020, validated 04 November 2020
Berec, L. (2020) More intense symptoms, more treatment, more drug-resistance: coevolution of virulence and drug-resistance. Peer Community in Evolutionary Biology, 100113. 10.24072/pci.evolbiol.100113
Recommendation
Mathematical models play an essential role in current evolutionary biology, and evolutionary epidemiology is not an exception [1]. While the issues of virulence evolution and drug-resistance evolution resonate in the literature for quite some time [2, 3], the study by Alizon [4] is one of a few that consider co-evolution of both these traits [5]. The idea behind this study is the following: treating individuals with more severe symptoms at a higher rate (which appears to be quite natural) leads to an appearance of virulent drug-resistant strains, via treatment failure. The author then shows that virulence in drug-resistant strains may face different selective pressures than in drug-sensitive strains and hence proceed at different rates. Hence, treatment itself modulates evolution of virulence. As one of the reviewers emphasizes, the present manuscript offers a mathematical view on why the resistant and more virulent strains can be selected in epidemics. Also, we both find important that the author highlights that the topic and results of this study can be attributed to public health policies and development of optimal treatment protocols [6].
Mathematical models are simplified representations of reality, created with a particular purpose. It can be simple as well as complex, but even simple models can produce relatively complex and knitted results. The art of modelling thus lies not only in developing a model, but also in interpreting and unknitting the results. And this is what Alizon [4] indeed does carefully and exhaustively. Using two contrasting theoretical approaches to study co-evolution, the Price equation approach to study short-term evolution and the adaptive dynamics approach to study long-term evolution, Alizon [4] shows that a positive correlation between the rate of treatment and infection severity causes virulence in drug-sensitive strains to decrease. Clearly, no single model can describe and explain an examined system in its entirety, and even this aspect of the work is taken seriously. Many possible extensions of the study are laid out, providing a wide opportunity to pursue this topic even further. Personally, I have had an opportunity to read many Alizon’s papers and use, teach or discuss many of his models and results. All, including the current one, keep high standard and pursue the field of theoretical (evolutionary) epidemiology.
References
[1] Gandon S, Day T, Metcalf JE, Grenfell BT (2016) Forecasting epidemiological and evolutionary dynamics of infectious diseases. Trends Ecol Evol 31: 776-788. doi: https://doi.org/10.1016/j.tree.2016.07.010
[2] Berngruber TW, Froissart R, Choisy M, Gandon S (2013) Evolution of virulence in emerging epidemics. PLoS Pathog 9(3): e1003209. doi: https://doi.org/10.1371/journal.ppat.1003209
[3] Spicknall IH, Foxman B, Marrs CF, Eisenberg JNS (2013) A modeling framework for the evolution and spread of antibiotic resistance: literature review and model categorization. Am J Epidemiol 178: 508-520. doi: https://doi.org/10.1093/aje/kwt017
[4] Alizon S (2020) Treating symptomatic infections and the co-evolution of virulence and drug resistance. bioRxiv, 2020.02.29.970905, ver. 3 peer-reviewed and recommended by PCI Evol Biol. doi: https://doi.org/10.1101/2020.02.29.970905
[5] Carval D, Ferriere R (2010) A unified model for the coevolution of resistance, tolerance, and virulence. Evolution 64: 2988–3009. doi: https://doi.org/10.1111/j.1558-5646.2010.01035.x
[6] Read AF, T Day, and S Huijben (2011). The evolution of drug resistance and the curious orthodoxy of aggressive chemotherapy. Proc Natl Acad Sci USA 108 Suppl 2, 10871–7. doi: https://doi.org/10.1073/pnas.1100299108
The recommender in charge of the evaluation of the article and the reviewers declared that they have no conflict of interest (as defined in the code of conduct of PCI) with the authors or with the content of the article. The authors declared that they comply with the PCI rule of having no financial conflicts of interest in relation to the content of the article.
Evaluation round #1
DOI or URL of the preprint: 10.1101/2020.02.29.970905
Author's Reply, 07 Oct 2020
Decision by Ludek Berec, posted 19 May 2020
Three reviewers evaluated the manuscript and all agree that the study is interesting. On the other hand, all raise a number of comments that require a revision of the manuscript. These comments vary and include a request to (1) provide a more detailed biological ground for the model, (2) perform some sensitivity analysis with respect to model parameters as well as to motivate the selected parameter values, (3) provide a deeper justification of the modelling choices. I agree with all these comments.
The central aspect of the study appears to be that more virulent infections are treated more. However, I miss any consistent part of the manuscript, such a subsection, that would discuss this issue at a reasonable depth. Information is provided here and there, but I cannot see any formula that would model this. Moreover, Figure S3A that appears to describe this is given only in the appendix, and its legend is quite poor; How the points are obtained? Is the dashed line a fit? I think a deeper discussion of this has to precede model formulation. I admit I do not fully agree with the general idea that more treatment of more virulent individuals generally means faster recovery. I can imagine that severely symptomatic individuals, despite receiving more treatment, may recover much later than individuals with mild symptoms and less treatment.
Furthermore, it seems the parameter rho is constant in simulations and does not depend on treatment level and symptoms severity. Is this realistic? Is not more likely that when more treatment is provided then there is more likely that treatment fails (or vice versa), so that rho cannot be considered constant?
Is seems the only result that is reflected in the abstract comes from Figure 3B. Honestly, I do not see the shown difference worth the merit given in the abstract and am curious how small changes in model parameters may change the curves. On the other hand, why results from adaptive dynamics are not reflected in the abstract?
Regarding adaptive dynamics, I am curious why calculations conserving evolutionary and convergence stability are not provided and a typical pairwise invasibility plot is not shown.
My final remark concerns the use of Price equation technique. As Figure 2 suggests, even at quite short temporal scale the approximation power of the Price equation system is quite poor, as the author himself admits, so I really do not see utility of it. Why the author keeps it here? I can imagine moving everything of it to an appendix and say it does not work well, but I regret not providing it in any form, but as it is now I doubt it is useful.
In summary, I also find the study interesting and useful, but at the same time think the author should revise the manuscript in order to present motivation, model formulation and results presentation in a more balanced way.
Reviewed by anonymous reviewer 1, 01 May 2020
Summary:
This article aims to mathematically describe a co-evolutionary dynamics of virulence and drug resistance of a pathogen in a population infected by drug-sensitive and drug-resistant strains. The proposed model assumes that there is a correlation between virulence and the rate at which infecteds receive treatment. Co-evolution of the pathogen virulence and drug resistance under the virulence-treatment relationship is investigated. My comments and questions are as follows.
I think the introduction section could benefit from adding specific examples of viral, bacterial or fungal infections to which the proposed hypothesis/model, that is, infected individuals with increased virulence receive treatment more quickly and their recovery is thus faster, could be applied.
The model 1a-1c assumes that all infecteds receive treatment though at different rates depending on the virulence of the strain type ‘i’. A fraction (1 - rhoi) recovers and a fraction rhoi transitions to the resistant state. Here, if I understand correctly, recovery occurs immediately upon treatment administration, is positively correlated with virulence (more virulent implies more rapid treatment and thus recovery) and if there is no treatment (gamma = 0), there is no recovery. I wonder if having the natural recovery of drug-resistant strain (traded-off with virulence) in the model might be reasonable since people can spontaneously recover from a large number of viral and bacterial infections. Also, the model assumes that all infecteds receive treatment; perhaps having treated and untreated classes would be interesting to incorporate and investigate. For the case of self-medication/prophylaxis, a paper here https://doi.org/10.1111/j.0014-3820.2005.tb01008.x might be worth looking at.
The model assumes that drug treatment directly affects recovery. I could imagine that there might be indirect effect of the drug through e.g. decreasing the infectious pathogen load/virulence and thus potentially accelerating recovery. Is that something that could be investigated via the model and affect the co-evolutionary dynamics?
In the relationships for virulence and transmission of the resistant strains, alphai^R = a*alpha and betai^R = a*beta, the assumption is that a >1 or 0 <= b < 1, so that drug-resistance results in higher virulence and decreased transmission. It might be useful to elaborate more on these assumptions and the choice of their values.
The trade-off between virulence and treatment rate (p. 19, Fig. S3 A) assumes a linear positive relationship between the two. I imagine that this relationship could be sigmoid so that those infected with low to mid-virulent pathogens would receive treatment at slower rates. I would be curious how this would affect the outcome.
On Fig. S3 A, do these points represent the values used in the simulations with multiple strains? Perhaps it would be good to have a more detailed description of the used methods also in the text so that one does not need to look into the R code.
In the equation S9c, rho is assumed to be a function of virulence. Is this assumption used anywhere else in the manuscript or simulations?
Reviewed by anonymous reviewer 3, 27 Apr 2020
The present manuscript offers a mathematical view on why the resistant and more virulent strain can be selected in epidemics. I have found interesting that the author highlighted the important aspect that this can be in particular attributed to public health policies.
Although I like and appreciate mathematics, I think it would be beneficial to give some ground for the performed simulations: for example, to adjust some values and time scale for a particular pathogen in mind. Otherwise, it is difficult to understand, e.g., what the time units for Fig.1 (is the x-axis in ages like for HIV or TB or in weeks like for influenza?). It would be also great to see more connection of the framework with real examples/diseases outlined in the introduction. Even though the discussion is quite detailed, I wish to see the same for Introduction section. In this respect, there is an imbalance between Introduction and Discussion sections.
Main remarks: L32: I have encountered a small contradiction in the framework. As the author writes about incubation period vs illness onset, but his main model does not include the "E" compartment. How the results would be changed or not changed if one would consider SEIR model instead of SIR model and how important the inclusion of the "E" compartment in view of the evolutionary dynamics? L63: I think that the examples make sense, but, e.g., TB is not modeled by a simple SIR model. In this view, the paragraph is more suited for introduction part. Then the author writes about parasites in L70, but all examples above except of Plasmodium falciparum are not parasites. Hence there is some mixture of terms, and it can be confusing.
Minor: L28: Would it be possible to clarify the term "pleiotropic action" for a general reader? L30: "the latter trait is usually binary rather than continuous." – the author does not explain much on why it is important. At the moment that sentence seems out of place. L32: I would advice to change the word order: the incubation period and the illness onset, because the former comes first. Introduction: I have noticed the gap in the literature dates, and it looks suspicious, because there should be some scientific works in the last few years. For example, Sylvain Gandon probably tackled some relevant problems, but it is just my guess. For example, the work Todd L. Parsons et al 2018 RSIF (doi:10.1098/rsif.2018.0135) looks relevant at least for the notion. L85: I would write "a disease-free equilibrium" instead of "a trivial state".
Reviewed by anonymous reviewer 2, 23 Apr 2020
This paper is an interesting addition to the literature on the interaction between epidemiological processes. The author states and then illustrates than differential treatment of individuals based on the virulence of their infecting pathogen strains can cause changes in the absolute and relative levels of virulence in the resistant and sensitive strains.
The analysis appears robust and the conclusions are interesting. The analysis of the impact of noise in transmission rates is appreciated, but a more general consideration of the robustness of the results to changes in parameter values would add to the manuscript, as if the effect occurs only in a small region of the parameter space (in this, admittedly, highly abstracted model), it is less likely to have large biological relevance in the real world.
On the topic of modelling drug resistance as a continuous trait, the author admits that that the inclusion would render the model unable to show the effect stated (due to modelling the modelling framework). While this is not an inditement of the work in this paper, it might be worth the author’s time to add to this section to describe ways that continuous modelling of virulence could be performed. Just off hand, it appears that this could be done using (for instance) something analogous to the integro-partial differential equation framework of Delgado-Eckert et al in The evolution of virulence in RNA viruses under a competition-colonization trade-off (https://arxiv.org/pdf/1001.3101.pdf), albeit at the cost of the simple mathematical formulation, that is a strength of this paper. Something to consider.
However, overall I enjoyed reading the paper and it seems like a valuable addition to the literature!
General notes:
Line 53 & 54: “by a drug resistant host” - it is the pathogen that is drug resistant, not the host
The section after equations 2a and 2b is confusing. It eventually becomes clear the author is using x-bar as a generic stand in for the weighted mean of any parameter in the equations, but this would be best stated explicitly, so the reader is not attempting to find and work out what trait x and y actually correspond to.
The equilibria are defined have no strain subscripts at line 84, but equilibria 2 and 3 are given with subscripts. Is this meant to imply that each strain persists with its own equilibrium level given by their own strain specific parameters? If so, this would be better explicitly stated.
Equation 4 - as far as I can see, the subscript m is not defined either in the text or the appendix. It first appears after the calculation of the eigenvalues in appendix A, but is not commented on. Its meaning should be explicitly stated.
Line below equation 4 - apparition is presumably mean to be appearance?
Line 89 - I assume the dichotomy being referred to is which of the eigenvalues dominates and therefore determines the fate of the mutant? But this should be clarified.
Line 148 - misspelling of average