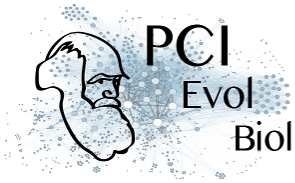
Detecting loci under natural selection from temporal genomic data of selfing populations
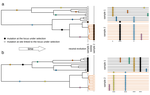
Power and limits of selection genome scans on temporal data from a selfing population
Abstract
Recommendation: posted 23 October 2020, validated 26 October 2020
Fumagalli, M. (2020) Detecting loci under natural selection from temporal genomic data of selfing populations. Peer Community in Evolutionary Biology, 100110. 10.24072/pci.evolbiol.100110
Recommendation
The observed levels of genomic diversity in contemporary populations are the result of changes imposed by several evolutionary processes. Among them, natural selection is known to dramatically shape the genetic diversity of loci associated with phenotypes which affect the fitness of carriers. As such, many efforts have been dedicated towards developing methods to detect signatures of natural selection from genomes of contemporary samples [1].
Recent technological advances made the generation of large-scale genomic data from temporal samples, either from experimental populations or historical or ancient samples, accessible to a wide scientific community [2]. Notably, temporal population genomic data allow for a direct observation and study of how, for instance, allele frequencies change through time in response to evolutionary stimuli. Such information can be exploited to detect loci under natural selection, either via mathematical modelling or by investigating empirical distributions [3].
However, most of current methods to detect selection from temporal genomic data have largely ignored selfing populations, despite the latter comprising a significant proportion of species with social and economic importance. Selfing changes genomic patterns by reducing the effective recombination rate, which makes distinguishing between neutral evolution and natural selection even more challenging than for the case of outcrossing populations [4]. Nevertheless, an outlier-approach based on temporal genomic data for the selfing Arabidopsis thaliana population revealed loci under selection [5].
This study suggested the promise of detecting selection for selfing populations and encouraged further investigations to test the power of selection scans under different mating systems.
To address this question, Navascués et al. [6] extended a previously proposed approach for temporal genome scan [7] to incorporate partial self-fertilization. In the original implementation [7], it is assumed that, under neutrality, all loci provide levels of genetic differentiation drawn from the same distribution. If some of the loci are under selection, such distribution should show heterogeneity. Navascués et al. [6] proposed a test for the homogeneity between loci-specific and genome-wide differentiation by deriving a null distribution of FST via simulations using SLiM [8]. After filtering for low-frequency variants and correct for multiple tests, authors derived a statistical test for selection and assess its power under a wide range of scenarios of selfing rate, selection coefficient, duration and type of selection [6].
The newly proposed test achieved good performance to distinguish between neutral and selected loci in most tested scenarios.
As expected, the test's performance significantly drops for scenarios of high selfing rates and selection from standing variation. Additionally, the probability to correctly detect selection decreases with increasing distance from the causal variant. Intriguingly, the test showed high power when the selected ancestral allele had an initial low frequency, and when the selected derived allele had a high initial frequency. When applied to a data set of around 1,000 SNPs from the highly selfing Medicago truncatula population, an annual plant of the legume family [9], the test did not provide any candidate loci under selection [6].
In summary, the detection of loci under selection in selfing populations is and largely remains a challenging task even when explictly account for the different mating system. However, recombination events that occurred before the selective pressure allow ancestral beneficial alleles to exhibit a detectable pattern of non-neutrality. As such, in partially selfing populations, the strength of the footprint of selection depends on several factors, mostly on the selfing rate, the time of onset and type of selection.
One major assumption of this study is that the model implies unstructured population and continuity between samples obtained from the same geographical location over time. As such assumptions are typically violated in real populations, further research into the effect of more complex demographic scenarios is desired to fully understand the power to detect selection in selfing populations. Furthermore, more power could be gained by including additional genomic information at each time point. In this context, recent approaches that make full use of genomic data based on deep learning [10] may contribute significantly towards this goal. Similarly, the effect of data filtering on the power to detect selection should be further explored, especially in the context of DNA resequencing experiments. These analyses will help elucidate the power offered by selection scans from temporal genomic data in selfing populations.
References
[1] Stern AJ, Nielsen R (2019) Detecting Natural Selection. In: Handbook of Statistical Genomics , pp. 397–40. John Wiley and Sons, Ltd. https://doi.org/10.1002/9781119487845.ch14
[2] Leonardi M, Librado P, Der Sarkissian C, Schubert M, Alfarhan AH, Alquraishi SA, Al-Rasheid KAS, Gamba C, Willerslev E, Orlando L (2017) Evolutionary Patterns and Processes: Lessons from Ancient DNA. Systematic Biology, 66, e1–e29. https://doi.org/10.1093/sysbio/syw059
[3] Dehasque M, Ávila‐Arcos MC, Díez‐del‐Molino D, Fumagalli M, Guschanski K, Lorenzen ED, Malaspinas A-S, Marques‐Bonet T, Martin MD, Murray GGR, Papadopulos AST, Therkildsen NO, Wegmann D, Dalén L, Foote AD (2020) Inference of natural selection from ancient DNA. Evolution Letters, 4, 94–108. https://doi.org/10.1002/evl3.165
[4] Vitalis R, Couvet D (2001) Two-locus identity probabilities and identity disequilibrium in a partially selfing subdivided population. Genetics Research, 77, 67–81. https://doi.org/10.1017/S0016672300004833
[5] Frachon L, Libourel C, Villoutreix R, Carrère S, Glorieux C, Huard-Chauveau C, Navascués M, Gay L, Vitalis R, Baron E, Amsellem L, Bouchez O, Vidal M, Le Corre V, Roby D, Bergelson J, Roux F (2017) Intermediate degrees of synergistic pleiotropy drive adaptive evolution in ecological time. Nature Ecology and Evolution, 1, 1551–1561. https://doi.org/10.1038/s41559-017-0297-1
[6] Navascués M, Becheler A, Gay L, Ronfort J, Loridon K, Vitalis R (2020) Power and limits of selection genome scans on temporal data from a selfing population. bioRxiv, 2020.05.06.080895, ver. 4 peer-reviewed and recommended by PCI Evol Biol. https://doi.org/10.1101/2020.05.06.080895
[7] Goldringer I, Bataillon T (2004) On the Distribution of Temporal Variations in Allele Frequency: Consequences for the Estimation of Effective Population Size and the Detection of Loci Undergoing Selection. Genetics, 168, 563–568. https://doi.org/10.1534/genetics.103.025908
[8] Messer PW (2013) SLiM: Simulating Evolution with Selection and Linkage. Genetics, 194, 1037–1039. https://doi.org/10.1534/genetics.113.152181
[9] Siol M, Prosperi JM, Bonnin I, Ronfort J (2008) How multilocus genotypic pattern helps to understand the history of selfing populations: a case study in Medicago truncatula. Heredity, 100, 517–525. https://doi.org/10.1038/hdy.2008.5
[10] Sanchez T, Cury J, Charpiat G, Jay F Deep learning for population size history inference: Design, comparison and combination with approximate Bayesian computation. Molecular Ecology Resources, n/a. https://doi.org/10.1111/1755-0998.13224
The recommender in charge of the evaluation of the article and the reviewers declared that they have no conflict of interest (as defined in the code of conduct of PCI) with the authors or with the content of the article. The authors declared that they comply with the PCI rule of having no financial conflicts of interest in relation to the content of the article.
Evaluation round #1
DOI or URL of the preprint: 10.1101/2020.05.06.080895
Version of the preprint: 2
Author's Reply, 08 Oct 2020
Decision by Matteo Fumagalli
, posted 08 Jul 2020
Dear Authors,
many thanks for your submission and please accept my apologies for the delay in processing your study which has now been reviewed by three experts in the field.
All reviewers and I agree that this manuscript is well written and present a solid piece of work. The study’s scope and implications are of wide interest as detecting adaptation in selfing species is an important but neglected topic in evolutionary genomics. The method presented herein is an extension of previous work on detecting selection from temporal data (sampled allele frequencies) for inbred/selfing species. The findings that selection from standing variation leaves a more evident genetic pattern than from de novo mutation in selfing species is a potential novel aspect which could be further tested empirically.
Before recommending this study, I encourage Authors to address the main points raised by reviewers which I summarise below.
The main point raised by all reviewers is on the assumption of no population structure. While Authors acknowledge and discuss this issue, I believe the study will greatly improved if Authors provide more intuition of how much population structure / discontinuity / metapopulations would affect their results (e.g. estimation of parameters and power to detect selection). I am not advocating for additional large-scale simulations but for more specific discussion on potential limitations for not including more complex but realistic scenarios (e.g. change of Ne, variation in recombination rate, linked selection on deleterious alleles).
The text on the methodology should be clarified, as pointed out by Reviewers. This is an important aspect to avoid readers having to extensively look at cited papers to understand the methodology. I also found it difficult to understand, for instance, when Authors used unlinked or linked SNPs in different analyses.
Another point raised by one Reviewer is how to evaluate the statistical uncertainty of parameters’ estimates. Likewise, there are some concerns on the use of the arbitrary threshold of 0.05 for minimum global MAF. The text should be either clarified or additional results varying this threshold should be presented.
I have an additional comment. I appreciate the discussion on how to design sequencing experiments in light of these results. I’d like to see future directions and ideas for improving the detection of selection for selfing to be elaborated a bit more carefully. For instance, Authors briefly mentioned ABC and as such I wonder whether Authors have more precise thoughts on which aspect of the methodology could be improved to achieve higher power (e.g. use of more features than single allele frequencies? Different inferential framework such as ABC or ML?). This doesn’t have to be too extensive tough.
I have some personal minor comments. Should the estimates of Ne on real data be presented in the results as first instance? As beneficial alleles are randomly assigned to a position, is there any border effect if such sites are too close to one of the extremities of the simulated region (e.g. for Fig. 3)? I appreciate that all scripts are provided but the documentation is rather thin and as such it is possible but unnecessarily laborious to replicate all analyses reported herein. Were the parameters of simulations chosen to match any organism of interest? I believe some reference that these values (mutation and recombination rate, Ne) are what expected in nature. Please provide a citation when introducing the equation Ne=(2-sigma)N/2.
Finally, please also address all minor issues raised and check your text carefully for typos as I was able to spot a few (e.g. “?.” on page 8, line 9).
Please do not hesitate to contact me in you need further clarification on any of these comments.
Additional requirements of the managing board:
As indicated in the 'How does it work?’ section and in the code of conduct, please make sure that:
-Data are available to readers, either in the text or through an open data repository such as Zenodo (free), Dryad or some other institutional repository. Data must be reusable, thus metadata or accompanying text must carefully describe the data.
-Details on quantitative analyses (e.g., data treatment and statistical scripts in R, bioinformatic pipeline scripts, etc.) and details concerning simulations (scripts, codes) are available to readers in the text, as appendices, or through an open data repository, such as Zenodo, Dryad or some other institutional repository. The scripts or codes must be carefully described so that they can be reused.
-Details on experimental procedures are available to readers in the text or as appendices.
-Authors have no financial conflict of interest relating to the article. The article must contain a "Conflict of interest disclosure" paragraph before the reference section containing this sentence: "The authors of this preprint declare that they have no financial conflict of interest with the content of this article." If appropriate, this disclosure may be completed by a sentence indicating that some of the authors are PCI recommenders: “XXX is one of the PCI XXX recommenders.”
Reviewed by anonymous reviewer 1, 02 Jul 2020
The manuscript of Navascués and colleagues describes a new method to detect regions of the genome under selection in partial selfing species using temporal data. The main methodological novelty comes from extending previous temporal methods to model genotype frequencies rather than allele frequencies, allowing to account for partial selfing. The authors performed a simulation study to validate and assess the power of their approach. Furthermore, they applied the method to a dataset with 1920 SNPs genotyped at 160 individuals from two time points of the selfing plant species Medicago truncatula. The simulation study results indicate that, as expected, the power to detect sweeps with genome scans in selfing species is limited. A promising result is that for partial selfing species, the proposed method has some power to detect sweeps. I find it particularly interesting and novel that in those cases, results indicate it is easier to detect selection from standing variation than from new mutations, contrary to what is expected for outcrossing populations. The authors interpret this result in terms of the age of mutations and historical recombination before the sweep. Even though the simulation study is not extensive (e.g. the effect of varying Ne and recombination rate was not considered), I think that the results are sufficient to reach their main conclusions.
Overall, the manuscript is well written and most of the material, methods and results are clear. Given the increased number of empirical datasets with time-series genetic data, and given that partial selfing occurs in many plant species, I think this is an interesting study for empiricists and also for theoreticians, and can promote further methodological developments. However, I have some technical concerns that can affect the conclusions and I think should be address before recommendation for PCI.
Main concerns:
One of the main conclusions is that in partial selfing species, it is easier to detect sweeps from standing variation. However, the authors applied a MAF filter, discarding sites where the average minor allele frequency between the two time points was less than 0.05. Could this conclusion might simply reflect the fact that sweeps from new mutations with initial frequency of 1/2Ne did not have enough time in 25 generation to reach an average frequency larger than 0.05? Another potential related problem is that the test is based on the single site distribution of FST under the null hypothesis of drift. It is known that the maximum value of FST estimators depends on the minor allele frequencies, and hence for rarer alleles we expect lower bounds for the maximum FST value (e.g. Jakobsson et al. 2013 Genetics doi:10.1534/genetics.112.144758). Can these results reflect such limitations with single site FST-based estimators? The authors justify the choice of the MAF filter based on deviations from a uniform distribution of p-values under the null hypothesis. However, they used an arbitraty threshold of 0.05. Given that it can the impact the detection of sweeps from new mutations, I think that the effects of such filtering options need to be better explored (e.g. MAF=1/2Ne, MAF=0.01).
The authors extend previous methods based on a two step approach, common in genome scans based on outlier loci detection. First, based on the entire dataset they estimate the effective population size (Ne) and selfing rate. Second, conditional on those estimates, they obtain the single site distribution of FST under neutrality and compute a p-value for each site. The authors use point estimates of Ne (based on FST) and of selfing (based on FIS) in the first step, ignoring the uncertainty on FST and FIS estimates. When analysing the real dataset from M. truncatula no evidence for sweeps was detected. The explanation was the very small Ne (~40) and a very large selfing rate (~0.97). How much uncertainty is there on the estimates of Ne and selfing in the real data? How would be the conclusion affected by accounting for the uncertainty? I think this needs to be further investigated, e.g. by considering simulations done with values within the range of the confidence interval for FST and FIS, which could be obtained with resampling methods.
Minor comments:
I have some doubts about the method that I think should be clarified in the main text:
a) Page 3. Null distribution of drift. Please clarify in the text that when you obtain the null distribution of FST the underlying assumption is that all SNPs are independent, and that there is no account for linkage disequilibrium in the null distribution. This might be particularly relevant for selfing species where we expect linkage disequilibrium can have a genome-wide extent.
b) Page 3. Typo in the posterior of the Dirichlet for the genotype frequencies? I think that you mean that you use as a prior for the genotype frequencies, which I denote by the vector gamma0, a uniform Dirichelt D(gamma0, 1). By using information on the genotype counts at time 0 (K0), you get the posterior for the genotype frequencies, which will be described by a Dirichlet D(gamma0, 1+K0). In the text it seems that the posterior is a Dirichlet D(K0, 1), which I think is incorrect. Please clarify.
c) Page 3. Equations of genotype frequencies. By using the FST and FIS estimators of Weir and Cockerham, I assume that the estimated FIS used to obtain the genotype frequencies (equations of Page 3) are based on the average FIS of the two time points. However, it is possible that the FIS would be different at time point 0 at the beginning of the sweep and at time point tau. Please clarify this in the text. Also, I am wondering whether the FIS estimated at time 0 and time tau could help distinguish the effect of selfing from the effect of selection, and hence increase the power of the selection test? Imagine that at time 0 you have a FIS0=0.9 and at time tau a FIStau=0.95. Assuming that the selfing rate was constant in the ancestral pop before the sweep, could the difference between the FIS estimates indicate the effects of selection on linked variation?
Page 3. Simulations. The simulations assume a constant Ne. Given that the Ne before the sweep will affect the recombination events and standing genetic variation, and given that you find that higher historical recombination results in higher power to detect selection, could your method have increased power by simulating a larger Ne before the sweep? Indeed, selection might occur when migrants colonize new environments (founder event) and for some species it is plausible that the Ne during the sweep is lower than before.
Page 5. Real data application. The description of the data is unclear – were the plants kept in the greenhouse since 1987? This would mean that several mutations could have occurred since then. I guess you mean that the seeds were maintained and then germinated. Please clarify that since the plants are annual you assume a generation time of 1 year. Is that correct?
Page 9, Arabidopsis thaliana results. “Based on the simulation results we present here, we can assume that this population has been adapting from standing variation over a short period of time (eight generations) rather than from many new mutations occurring during (or shortly before) the studied period.” Where do these results come from? I could not find the description of the data for Arabidopsis and the eight generations. Does this refer to M. truncatula results? Please clarify.
Fig. S2 shows the uniform distribution of p-values without selfing. What is the distribution of p-values with selfing? This should just be a re-scaling in Ne, but I think it would be important to see if with selfing the p-values also follow a uniform distribution.
Fig S5 legend: Typo? Unlcear what is the boxplot mentioned in the legend
Fig S7 legend: Typo. Please use symbol sigma rather than “sigma”.
There are a few other typos, especially in the results and discussion.
Reviewed by anonymous reviewer 2, 06 Jul 2020
In their manuscript titled ‘Power and limits of selection genome scans on temporal data from a selfing population’, Navascués et al. present a method by which the inference of selection from temporally spaced samples can be used when the focal species reproduces at least in part through selfing. The utility in allowing for reproductive systems which aren’t entirely outcrossing into the inference of selection with temporal sampled allele frequency data is clearly useful given there are so many biological systems that deviate from this expectation. My review is less detailed than I’d generally provide due to some unexpected time constraints. Nonetheless I would like to point out a few general concerns that I think would be useful for the authors to consider in order to improve the utility of the presented approach.
1) I have a general concern for the manuscript in how there seems to be a misspecification of what a ‘population’ is, and so while the intent is to suggest that increases in selfing will generate problems for inferring selection with temporal sampling, one would expect such to be the case in almost any situation in which a misspecification of this sort arises. If it’s not just a problem with misspecification, could one then expect the method to perform well in continuous and discrete population structures? If the former is the case, then the utility of the approach is quite a bit larger than the present manuscript’s focus. 2) The authors suggest that limited, or ‘effective’, recombination results from selfing, but then this is the same effect that arises as the result of spatial population structure. So while population structure certainly increases gametic LD, it’s not for a lack of recombination, and it’s the same for the case of selfing. This then provides a reiteration of point 1) above. 3) It is generally assumed that selfing, or a lack of outcrossing, is invariably a process that limits adaptation. I guess I can generally agree with this point, in that at least empirical literature has provided limited evidence to the contrary. But then I wonder what role variation in distinct ‘lineages’ carrying particular ‘ancenstral advantageous alleles at low frequency’ which then ‘leave the strongest local signal’ might again be an issue with misidentifying where and how selection is acting, thereby driving a disproportionate deviation from biological reality? This point concerns how beneficial alleles are considered while the next point considers deleterious alleles. 4) The effects of selection on linked sites is clearly important in understanding the distribution of genetic diversity across the genome. In a selfing species this might actually be even more important. Point 3) concerned the role of beneficial alleles but opposite might be even more important for general application of this method as there probably exists a general pattern of increased genetic load with increased selfing (though factors like Ne and Fst will clearly play a role in modulating just how much load exists). Given just how much constraint to positive selection which might arise through the effects of linked selection on deleterious alleles with high selfing/gametic LD I think it’s important to include this factor into the simulation approach. To not do so would certainly limit the degree to which the methodological advance might find direct use in natural systems.
Reviewed by Christian Huber, 16 Jun 2020
I enjoyed reading the manuscript by Navascués et al. They provide extensive simulations to investigate the power to detect directional selection from time-series data of genetic variants in an inbreeding/selfing population. The assumptions are that there is no population structure and that effective population size (Ne) and inbreeding coefficient (Fis) can be well estimated from the data and do not change over time. The test statistic is single-locus Fst between populations from two different time points, and the null distribution is derived from simulations of unlinked loci assuming the genome-wide estimates of Ne and Fis. The performance of the method is then tested on simulated data with recombination and selfing, and on real data from Medicago truncatula.
The software for deriving the null distribution is a simple extension of a previously published method and now accounts for the effect of inbreeding on genotype frequencies. The software is freely available and I was able to compile and use it on my computer.
I don't have any major concerns. The authors provide useful qualitative and quantitative insights. Below are a few comments that might improve the paper:
1) Maybe cite and relate the results to the recent paper by Hartfield and Bataillon (G3, 2020) on sweep patterns in selfing populations, e.g. regarding the size of the sweep in inbreeding populations for selection on new mutations vs. standing variation.
2) The description of the new method was somewhat hard to follow and I had to look up the original paper by Frachon et al. (2017) to understand it. I think it could be improved, for example by explicitly providing the steps for computing the significance of an Fst value at a specific locus.
3) The biggest assumption of the method, most likely violated in most selfing plant populations, is the assumptions of no population structure. This is discussed, but I wonder if the authors could provide more intuition on how such structure would affect their parameter estimation (Ne, Fis) and in general the performance of the method.