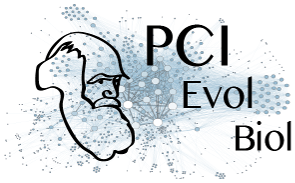
Be careful when studying selection based on polygenic score overdispersion
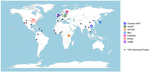
How robust are cross-population signatures of polygenic adaptation in humans?
Abstract
Recommendation: posted 03 April 2021, validated 06 April 2021
Günther, T. (2021) Be careful when studying selection based on polygenic score overdispersion. Peer Community in Evolutionary Biology, 100125. https://doi.org/10.24072/pci.evolbiol.100125
Recommendation
The advent of genome-wide association studies (GWAS) has been a great promise for our understanding of the connection between genotype and phenotype. Today, the NHGRI-EBI GWAS catalog contains 251,401 associations from 4,961 studies (1). This wealth of studies has also generated interest to use the summary statistics beyond the few top hits in order to make predictions for individuals without known phenotype, e.g. to predict polygenic risk scores or to study polygenic selection by comparing different groups. For instance, polygenic selection acting on the most studied polygenic trait, height, has been subject to multiple studies during the past decade (e.g. 2–6). They detected north-south gradients in Europe which were consistent with expectations. However, their GWAS summary statistics were based on the GIANT consortium data set, a meta-analysis of GWAS conducted in different European cohorts (7,8). The availability of large data sets with less stratification such as the UK Biobank (9) has led to a re-evaluation of those results. The nature of the GIANT consortium data set was realized to represent a potential problem for studies of polygenic adaptation which led several of the authors of the original articles to caution against the interpretations of polygenic selection on height (10,11). This was a great example on how the scientific community assessed their own earlier results in a critical way as more data became available. At the same time it left the question whether there is detectable polygenic selection separating populations more open than ever.
Generally, recent years have seen several articles critically assessing the portability of GWAS results and risk score predictions to other populations (12–14). Refoyo-Martínez et al. (15) are now presenting a systematic assessment on the robustness of cross-population signatures of polygenic adaptation in humans. They compiled GWAS results for complex traits which have been studied in more than one cohort and then use allele frequencies from the 1000 Genomes Project data (16) set to detect signals of polygenic score overdispersion. As the source for the allele frequencies is kept the same across all tests, differences between the signals must be caused by the underlying GWAS. The results are concerning as the level of overdispersion largely depends on the choice of GWAS cohort. Cohorts with homogenous ancestries show little to no overdispersion compared to cohorts of mixed ancestries such as meta-analyses. It appears that the meta-analyses fail to fully account for stratification in their data sets.
The authors based most of their analyses on the heavily studied trait height. Additionally, they use educational attainment (measured as the number of school years of an individual) as an example. This choice was due to the potential over- or misinterpretation of results by the media, the general public and by far right hate groups. Such traits are potentially confounded by unaccounted cultural and socio-economic factors. Showing that previous results about polygenic selection on educational attainment are not robust is an important result that needs to be communicated well. This forms a great example for everyone working in human genomics. We need to be aware that our results can sometimes be misinterpreted. And we need to make an effort to write our papers and communicate our results in a way that is honest about the limitations of our research and that prevents the misuse of our results by hate groups.
This article represents an important contribution to the field. It is cruicial to be aware of potential methodological biases and technical artifacts. Future studies of polygenic adaptation need to be cautious with their interpretations of polygenic score overdispersion. A recommendation would be to use GWAS results obtained in homogenous cohorts. But even if different biobank-scale cohorts of homogeneous ancestry are employed, there will always be some remaining risk of unaccounted stratification. These conclusions may seem sobering but they are part of the scientific process. We need additional controls and new, different methods than polygenic score overdispersion for assessing polygenic selection. Last year also saw the presentation of a novel approach using sequence data and GWAS summary statistics to detect directional selection on a polygenic trait (17). This new method appears to be robust to bias stemming from stratification in the GWAS cohort as well as other confounding factors. Such new developments show light at the end of the tunnel for the use of GWAS summary statistics in the study of polygenic adaptation.
References
1. Buniello A, MacArthur JAL, Cerezo M, Harris LW, Hayhurst J, Malangone C, et al. The NHGRI-EBI GWAS Catalog of published genome-wide association studies, targeted arrays and summary statistics 2019. Nucleic Acids Research. 2019 Jan 8;47(D1):D1005–12. doi: https://doi.org/10.1093/nar/gky1120
2. Turchin MC, Chiang CW, Palmer CD, Sankararaman S, Reich D, Hirschhorn JN. Evidence of widespread selection on standing variation in Europe at height-associated SNPs. Nature Genetics. 2012 Sep;44(9):1015–9. doi: https://doi.org/10.1038/ng.2368
3. Berg JJ, Coop G. A Population Genetic Signal of Polygenic Adaptation. PLOS Genetics. 2014 Aug 7;10(8):e1004412. doi: https://doi.org/10.1371/journal.pgen.1004412
4. Robinson MR, Hemani G, Medina-Gomez C, Mezzavilla M, Esko T, Shakhbazov K, et al. Population genetic differentiation of height and body mass index across Europe. Nature Genetics. 2015 Nov;47(11):1357–62. doi: https://doi.org/10.1038/ng.3401
5. Mathieson I, Lazaridis I, Rohland N, Mallick S, Patterson N, Roodenberg SA, et al. Genome-wide patterns of selection in 230 ancient Eurasians. Nature. 2015 Dec;528(7583):499–503. doi: https://doi.org/10.1038/nature16152
6. Racimo F, Berg JJ, Pickrell JK. Detecting polygenic adaptation in admixture graphs. Genetics. 2018. Arp;208(4):1565–1584. doi: https://doi.org/10.1534/genetics.117.300489
7. Lango Allen H, Estrada K, Lettre G, Berndt SI, Weedon MN, Rivadeneira F, et al. Hundreds of variants clustered in genomic loci and biological pathways affect human height. Nature. 2010 Oct;467(7317):832–8. doi: https://doi.org/10.1038/nature09410
8. Wood AR, Esko T, Yang J, Vedantam S, Pers TH, Gustafsson S, et al. Defining the role of common variation in the genomic and biological architecture of adult human height. Nat Genet. 2014 Nov;46(11):1173–86. doi: https://doi.org/10.1038/ng.3097
9. Bycroft C, Freeman C, Petkova D, Band G, Elliott LT, Sharp K, et al. The UK Biobank resource with deep phenotyping and genomic data. Nature. 2018 Oct;562(7726):203–9. doi: https://doi.org/10.1038/s41586-018-0579-z
10. Berg JJ, Harpak A, Sinnott-Armstrong N, Joergensen AM, Mostafavi H, Field Y, et al. Reduced signal for polygenic adaptation of height in UK Biobank. eLife. 2019 Mar 21;8:e39725. doi: https://doi.org/10.7554/eLife.39725
11. Sohail M, Maier RM, Ganna A, Bloemendal A, Martin AR, Turchin MC, et al. Polygenic adaptation on height is overestimated due to uncorrected stratification in genome-wide association studies. eLife. 2019 Mar 21;8:e39702. doi: https://doi.org/10.7554/eLife.39702
12. Martin AR, Kanai M, Kamatani Y, Okada Y, Neale BM, Daly MJ. Clinical use of current polygenic risk scores may exacerbate health disparities. Nature Genetics. 2019 Apr;51(4):584–91. doi: https://doi.org/10.1038/s41588-019-0379-x
13. Bitarello BD, Mathieson I. Polygenic Scores for Height in Admixed Populations. G3: Genes, Genomes, Genetics. 2020 Nov 1;10(11):4027–36. doi: https://doi.org/10.1534/g3.120.401658
14. Uricchio LH, Kitano HC, Gusev A, Zaitlen NA. An evolutionary compass for detecting signals of polygenic selection and mutational bias. Evolution Letters. 2019;3(1):69–79. doi: https://doi.org/10.1002/evl3.97
15. Refoyo-Martínez A, Liu S, Jørgensen AM, Jin X, Albrechtsen A, Martin AR, Racimo F. How robust are cross-population signatures of polygenic adaptation in humans? bioRxiv, 2021, 2020.07.13.200030, version 5 peer-reviewed and recommended by Peer community in Evolutionary Biology. doi: https://doi.org/10.1101/2020.07.13.200030
16. Auton A, Abecasis GR, Altshuler DM, Durbin RM, Abecasis GR, Bentley DR, et al. A global reference for human genetic variation. Nature. 2015 Sep 30;526(7571):68–74. doi: https://doi.org/10.1038/nature15393
17. Stern AJ, Speidel L, Zaitlen NA, Nielsen R. Disentangling selection on genetically correlated polygenic traits using whole-genome genealogies. bioRxiv. 2020 May 8;2020.05.07.083402. doi: https://doi.org/10.1101/2020.05.07.083402
The recommender in charge of the evaluation of the article and the reviewers declared that they have no conflict of interest (as defined in the code of conduct of PCI) with the authors or with the content of the article. The authors declared that they comply with the PCI rule of having no financial conflicts of interest in relation to the content of the article.
Evaluation round #2
DOI or URL of the preprint: https://doi.org/10.1101/2020.07.13.200030
Version of the preprint: https://www.biorxiv.org/content/10.1101/2020.07.13.200030v3.full.pdf+html
Author's Reply, 01 Mar 2021
Decision by Torsten Günther
, posted 22 Feb 2021
Thank you very much for the revised version of the preprint. The reviewers and myself are very happy with the way you have addressed the comments from the previous round. There are still some minor edits to be done before we can start the recommendation process.
Reviewed by Lawrence Uricchio, 17 Feb 2021
I'm grateful to the authors for their very detailed reply. The manuscript is clear and concise in synthesizing quite a lot of complex results, and I found it quite interesting to read. I have a few very minor suggestions, detailed below, and I will defer to the authors as to whether/how to make any further changes to the manuscript in response to these suggestions.
-The authors write "We obtained GWAS summary statistics from five large-scale biobanks a GWAS meta-analysis and a mega-analysis." This sentence seems like it needs a comma or two
-The authors wrote "Because we are using the exact same population panels to obtain population allele frequencies in all tests, the source of the inconsistencies must necessarily come from differences in the effect size estimates in the different GWAS" It might help to clarify in this line that the set of SNPs also differs between most of the comparisons performed, as the authors have mentioned elsewhere.
-The authors write "This suggests differences in scores are likely not driven by a biological signal and are instead driven by population stratification in GIANT and/or PAGE." I'm not sure I understand this sentence fully. I agree that the direction of the polygenic score difference between populations computed from estimated effects should be correlated with the "true" score difference between populations, but because these polygenic scores are computed from a subset of putatively causal SNPs that explain only a small percentage of the heritability, it seems like there is no guarantee that the estimated score difference between populations will be consistent in direction between studies, even if the differences are due to unbiased effect size estimates at true causal alleles. E.g., if we had only a small number of estimated effect sizes, one could easily infer a positive score difference between population A and B when the true difference using all of the unobserved effect sizes would be negative. I'm happy to be corrected here if I am misunderstanding the authors' point.
-"While modeling the individual effect of each of these on the inflation of the QX statistic is beyond the scope of this study, we note that all of these factors may be influencing the differences we observe among score sets." It might make sense to refer back to the partial attenuation of score differences reported when using a single set of SNPs here (i.e. Figures S8/S9).
Sincerely, Lawrence Uricchio
https://doi.org/10.24072/pci.evolbiol.100254.rev21Reviewed by Mashaal Sohail, 19 Jan 2021
Dear authors,
I commend you on the new analyses, revisions to the text, and clarification. All my comments have been addressed, and the manuscript will stand as an important reference for many researchers in the field.
If the authors see value in this as well, I think it would be useful to see analyses like Figure 5 of population stratification for educational attainment as well to help interpret their newly added section comparing polygenic scores for this trait using different GWAS. This would of course be for the GWAS that they considered for this trait, and along a few axes of potential stratification (as they present a global analysis). I suggest this as, as a reader, an open question that remains for me with their new section is how much are the different GWAS that show different over dispersion values afflicted by stratification or not for this trait. That, is stratification as much a concern for educational attainment as they have shown it is for height?
Sincerely,
Mashaal Sohail
https://doi.org/10.24072/pci.evolbiol.100254.rev22Reviewed by Barbara Bitarello, 19 Feb 2021
This revised version of the manuscript "How robust are cross-population signatures of polygenic adaptation in humans?" addressed, in my opinion, all points raised by the reviewers in the previous round of reviews. It addresses the important topic of how interpretations of polygenic risk score dispersion across populations can be misleading. The authors look at many traits for which there is at least two other GWAS apart from the UKBB, but they focus mostly on height and educational attainment - the former because of having multiple publicly available GWAS and previous claims for evidence of polygenic selection in Europe and the latter due to its high interest in the media and great potential for misappropriation by far-right hate groups.
The authors show unequivocally that measures of polygenic risk score dispersion across populations:
1) depend on the set of SNPs used
2) depends on whether SNPs were ascertained (chosen for the PRS) in the large single ancestry cohort (aka UKBB in this study) or in the non-UKBB ancestries, regardless of which effect sizes are subsequently used
3) depends on how homogenous (ancestry-wise) the GWAS is - the more homogenous, the less overdispersion is observed
4) and whether the inference comes from a single GWAS vs meta-analysis (even of a single ancestry). To illustrate this
the point they split the UKBB into sub-cohorts and then meta-analyzed them, finding increased overdispersion of PRS mimicking that seen for GIANT and not seen in the UKBB single-cohort analysis
5) is inconsistent for educational attainment depending on the GWAS chosen, resulting in different ancestries having higher PRS values
6) in brief, it is increased by having multiple ancestries in the GWAS (e.g. the PAGE study) and/or multiple sub-cohorts composing a meta-analysis (e.g. GIANT)
7) the patterns seen for meta-analyses are independent of the method (standard error or sample size based)
Their findings strongly suggest that population stratification in GIANT and PAEGE are driving these findings, although the possibility remains that more diverse cohorts such as PAGE are better at capturing true biological signals that are overcorrected for in the UK Biobank.
Overall I think this is a great contribution to the field and an important methodological manuscript on the caveats and biases involved in polygenic selection studies/interpretation.
The authors used publicly available data and provided a link for a repository containing the scripts needed to reproduce this analysis.
Finally, I want to enthusiastically commend the authors for plainly pointing out how this kind of study has enormous potential for misinterpretation (e.g. educational attainment). I would like to see this become way more prevalent in the literature.
Very minor comments:
- for clarity: please make sure to emphasize which 'score' authors are talking about in different parts of the paper: the PRS or the Qx.
- for the educational attainment GWASs, how did the authors handle the sample overlap?
- table S2: Since the N for GIANT is variable across positions, authors should clarify that this value is the maximum N.
- tables S5-S6: I imagine these refer to height but the captions should say it.
Sincerely,
Bárbara D. Bitarello
Evaluation round #1
DOI or URL of the preprint: https://doi.org/10.1101/2020.07.13.200030
Author's Reply, 13 Jan 2021
Decision by Torsten Günther
, posted 24 Sep 2020
Thank you very much for your patience. Your preprint has been seen by three expert reviewers. They all provide a very detailed list of reasonable comments but no major criticism, so I think it should be possible to address them in a revised version of the manuscript.
I am looking forward to receiving your revised preprint.
Additional requirements of the managing board:
As indicated in the 'How does it work?’ section and in the code of conduct, please make sure that:
-Data are available to readers, either in the text or through an open data repository such as Zenodo (free), Dryad or some other institutional repository. Data must be reusable, thus metadata or accompanying text must carefully describe the data.
-Details on quantitative analyses (e.g., data treatment and statistical scripts in R, bioinformatic pipeline scripts, etc.) and details concerning simulations (scripts, codes) are available to readers in the text, as appendices, or through an open data repository, such as Zenodo, Dryad or some other institutional repository. The scripts or codes must be carefully described so that they can be reused.
-Details on experimental procedures are available to readers in the text or as appendices.
-Authors have no financial conflict of interest relating to the article. The article must contain a "Conflict of interest disclosure" paragraph before the reference section containing this sentence: "The authors of this preprint declare that they have no financial conflict of interest with the content of this article." If appropriate, this disclosure may be completed by a sentence indicating that some of the authors are PCI recommenders: “XXX is one of the PCI XXX recommenders.”
Reviewed by Lawrence Uricchio, 10 Sep 2020
Reviewed by Mashaal Sohail, 24 Sep 2020
Reviewed by anonymous reviewer 1, 09 Sep 2020
I think this paper is clearly written, well thought out, and brings a considerable contribution to the field. There are many challenges in interpreting differences in polygenic risk score differences across ancestries and cohorts, and this study addresses important points.
Introduction:
Second paragraph: it is true that there were issues with the simulations in Martin et al. 20219, and it is good that the authors mentioned that. However, there are both theoretical predictions (Wang et al. 2020) and empirical evidence (Marnetto et al. 2020, Bitarello & Mathieson 2020) that PRS portability is and should be low in the current state of GWAS diversity. Perhaps it would be nice to mention that as well.
Methods:
Is there evidence the PUR actually roughly matches “Latin American” frequencies? I.E, does it match Latin -Americans other than PUR better than other populations?
It should be mentioned that LD blocks (Berisa & Pickrell) are only available for European and African ancestries, correct?
Page 6: "a minor allele frequency (MAF) < 5% globally," Is this a typo? should it be > 5% globally?
Page 7: "7 standard deviations away from the first six PCs in a PCA of the set." Is this criterion based on some other publication, or some another analysis in this paper? It would be nice to see a justification for this particular filter.
Results
Page 7: "we also computed P-values using two randomization schemes: one is based on randomizing the effect size estimates of the trait-associated SNPs, while the other" Randomizing the signal, but not the actual magnitude of the effect, correct?
Page 7: "In general, we observe little notable differences in P-values when using the three schemes, although the sign-randomization scheme is sometimes inconsistent with the other two" Perhaps because the patterns are preserved, since all variants have their signs inverted, but not the magnitude of the effect size?
Page 8, second paragraph: the sentence starting with "indeed" needs to be revised.
Page 9, last paragraph: while this is a very nice (and informative) approach, don’t the GIANT cohort also have different sample sizes? That is not being emulated here and should be mentioned in the discussion.
https://doi.org/10.24072/pci.evolbiol.100254.rev13