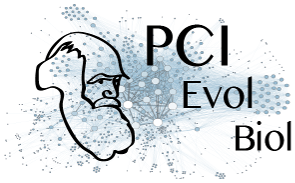
Sexual selection goes dynamic
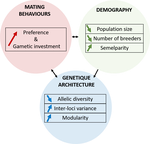
A demogenetic agent based model for the evolution of traits and genome architecture under sexual selection
Abstract
Recommendation: posted 21 October 2020, validated 18 November 2020
Greenfield, M. (2020) Sexual selection goes dynamic. Peer Community in Evolutionary Biology, 100112. https://doi.org/10.24072/pci.evolbiol.100112
Recommendation
150 years after Darwin published ‘Descent of man and selection in relation to sex’ (Darwin, 1871), the evolutionary mechanism that he laid out in his treatise continues to fascinate us. Sexual selection is responsible for some of the most spectacular traits among animals, and plants, and it appeals to our interest in all things reproductive and sexual (Bell, 1982). In addition, sexual selection poses some of the more intractable problems in evolutionary biology: Its realm encompasses traits that are subject to markedly different selection pressures, particularly when distinct, yet associated, traits tend to be associated with males, e.g. courtship signals, and with females, e.g. preferences (cf. Ah-King & Ahnesjo, 2013). While separate, such traits cannot evolve independently of each other (Arnqvist & Rowe, 2005), and complex feedback loops and correlations between them are predicted (Greenfield et al., 2014). Traditionally, sexual selection has been modelled under simplifying assumptions, and quantitative genetic approaches that avoided evolutionary dynamics have prevailed. New computing methods may be able to free the field from these constraints, and a trio of theoreticians (Chevalier, De Coligny & Labonne 2020) describe here a novel application of a ‘demo-genetic agent (or individual) based model’, a mouthful hereafter termed DG-ABM, for arriving at a holistic picture of the sexual selection trajectory. The application is built on the premise that traits, e.g. courtship, preference, gamete investment, competitiveness for mates, can influence the genetic architecture, e.g. correlations, of those traits. In turn, the genetic architecture can influence the expression and evolvability of the traits. Much of this influence occurs via demographic features, i.e. social environment, generated by behavioral interactions during sexual advertisement, courtship, mate guarding, parental care, post-mating dispersal, etc.
The authors provide a lengthy verbal description of their model, specifying the genomic and behavioral parameters that can be set and how a ‘run’ may be initialized. There is a link to an internet site where users can then enter their own parameter values and begin exploring hypotheses. Back in the article several simulations illustrate simple tests; e.g. how gamete investment and preference jointly evolve given certain survival costs. One obvious test would have been the preference – courtship genetic correlation that represents the core of Fisherian runaway selection, and it is regrettable that it was not examined under a range of demographic parameters. As presented the author’s DG-ABM appears particularly geared toward mating systems in ‘higher’ vertebrates, where couples form during a discrete mating season and are responsible for most reproduction. It is not clear how applicable the model could be to a full range of mating systems and nuances, including those in arthropods and other invertebrates as well as plants.
What is the likely value of the DG-ABM for sexual selection researchers? We will not be able to evaluate its potential impact until readers with specialized understanding of a question and taxon begin exploring and comparing their results with prior expectations. Of course, lack of congruence with earlier predictions would not invalidate the model. Hopefully, some of these specialists will have opportunities for comparing results with pertinent empirical data.
References
Ah-King, M. and Ahnesjo, I. 2013. The ‘sex role’ concept: An overview and evaluation Evolutionary Biology, 40, 461-470. doi: https://doi.org/10.1007/s11692-013-9226-7
Arnqvist, G. and Rowe, L. 2005. Sexual Conflict. Princeton University Press, Princeton. doi: https://doi.org/10.1515/9781400850600
Bell, G. 1982. The Masterpiece of Nature: The Evolution and Genetics of Sexuality. University of California Press, Berkeley.
Chevalier, L., De Coligny, F. and Labonne, J. (2020) A demogenetic individual based model for the evolution of traits and genome architecture under sexual selection. bioRxiv, 2020.04.01.014514, ver. 4 peer-reviewed and recommended by PCI Evol Biol. doi: https://doi.org/10.1101/2020.04.01.014514
Darwin, C. 1871. The Descent of Man and Selection in Relation to Sex. J. Murray, London.
Greenfield, M.D., Alem, S., Limousin, D. and Bailey, N.W. 2014. The dilemma of Fisherian sexual selection: Mate choice for indirect benefits despite rarity and overall weakness of trait-preference genetic correlation. Evolution, 68, 3524-3536. doi: https://doi.org/10.1111/evo.12542
The recommender in charge of the evaluation of the article and the reviewers declared that they have no conflict of interest (as defined in the code of conduct of PCI) with the authors or with the content of the article. The authors declared that they comply with the PCI rule of having no financial conflicts of interest in relation to the content of the article.
Evaluation round #2
DOI or URL of the preprint: https://www.biorxiv.org/content/10.1101/2020.04.01.014514v1
Version of the preprint: 1
Author's Reply, 14 Oct 2020
Decision by Michael D Greenfield, posted 19 Aug 2020
Reviewed by Frédéric Guillaume
, 17 Aug 2020
I am mostly OK with those pre-revision comments and suggest that the authors revise their manuscript accordingly.
However, I am stressing that the authors should make clear how their approach it different from current approaches in other simulation software. They should also be extra careful to clarify the generality of their genetic analysis approach and the link to outstanding questions in evolutionary quantitative genetics or population genetics. This is necessary to clarify the audience of the software. I would like the authors to answer two questions: Who is going to use your product ? and for what purpose? To me, the work described has two purposes: the individual-based simulation, and the analysis of allelic correlations with a bootstrap method. Could a user use the software to perform the analysis only? Is there interest in having such genetic analysis as a standalone method? If so, you might be better off separating the two aspects in two distinct products/publications.
About the statistical method. Please clarify how this is different from analysis of pattern of linkage disequilibrium (LD) along the genome.
https://doi.org/10.24072/pci.evolbiol.100236.rev21Evaluation round #1
DOI or URL of the preprint: https://www.biorxiv.org/content/10.1101/2020.04.01.014514v1
Author's Reply, 27 Jul 2020
Comment of the managing board: the following is a direct answer of the authors to one of the reviewer.
Dear Dr. Guillaume.
First and foremost, thank you for this detailed review of our manuscript, and all the work accomplished. We apologize for the delay in our response. We plan to resubmit an improved version of the document to PCI Evol Biol in the coming month. We believe the paper can be significantly improved through clarification, following your advice, in a few general directions, and we would like to have your opinion on the following choices:
First, by better positioning our work: As noted, we failed to position our paper between other genetically explicit IBMs, the main particularity of our model is the focus on behavioral interactions between individuals in the context of sexual selection. In other genetically explicit models, the selective pressure on traits is a priori defined and therefore do not emerge from inter-individual interactions, and from the trade-off between traits. By contrast, in our approach, traits values directly influence mating behaviors and survival of individuals, allowing the selection pressure to change with the distribution of the traits values in the population, organisms thus modifying their social environment and being able to respond dynamically to this change.
Second, by stating more clearly our genetic formalism: Indeed, we noticed that you interpreted several aspects of our model following a quantitative genetics point of view. Our model is not based on quantitative genetics, and therefore several of your comments do not apply. Still, the fault is ours for not having described more clearly the genetic approach (allelic model). There is indeed no neutral mutation (or allele) in our model, and the genetic value of zero for an allele has an effect on the trait(s) expressed. This choice is motivated by the fact that our model directly integrates a trade-off, wherein the size of the trait has a cost on survival (so in terms of natural selection). Additionally, we used the Beta distribution to let the user simulate and approximate different possible distribution of effects for mutations: uniform distribution, biased distribution toward small or large mutations (at least relative to survival), or possibly a Gaussian-like distribution. Besides, we should have clarified our measures of genetic architecture ( “polygeny” and “pleiotropy,” see further).
We also are currently investigating how we could compare the output of our model (accounting for genetic variance, population stability – or the lack thereof – effective number of breeders) to theoretical expectation under the assumptions of quantitative genetic (Turelli, 1984; Burger, 2000) as you suggested. Namely, we want to investigate the relationship between the effective number of breeders in the population, the selection regime and strength, and the genetic variance. To do so, we will focus on the random mating scenario (no preference no competition) and compare the genetic variance of gametic investment with theoretical expectation (by approximating the effective number of breeders in the population, the selection regime, and strength). We hope this example can answer your concern.
Lastly, we noted that the manuscript appeared as ambivalent (between the description of a modelling approach and analysis of evolutionary situations), and we agree that it should somehow be rebalanced. Our opinion is that the new version of the manuscript should indeed focus more on the presentation of the model and on its perks/ interests / benefits compared to the existing literature. Our general philosophy would therefore to strip the paper of part of the analyses (regarding the effect of physical structure of the genome on the evolution), re-write some portions of the presentation (namely how to install, launch and handle the simulations), and include a paragraph dedicated to the specifics of our approach in relation to existing models.
We noted your concern regarding our vision of the genetic architecture. We agree that what we termed as “polygeny” was in fact a description of the distribution of effect sizes, and that we could call it “oligogenicity”: to go further in that direction, we are currently integrating a measure of the variance between loci (centered on the mean of the population), so to distinguish simulations where all genes have approximately the same contribution to the trait value versus simulations where loci contribute unequally to the trait value. This, for us, is of interest, because it shed some light on how natural and sexual selection shapes the contribution of various loci to reach an evolutionary pseudo-equilibrium.
Regarding “pleiotropy” in our model, we acknowledge that it can be more accurately called “correlation of allelic effects”. However, we stress the interest of looking at this correlation at the gene level, because it can impact the evolution beyond what it can be predicted by the G- matrix. The G-matrix sums up all necessary information to describe correlation between traits at condition that the distribution of allele effects is normally spread around mean values, and that traits are coded by an infinitesimal number of loci with small additive effects (which is not mandatory in our model). By contrast we here propose to study the spatial organisation of allelic values in the genome, and therefore investigate pattern of correlation at the gene level. Our bootstrap approach accounts for the mutations retained in the population versus what would be obtained by randomly sampling into the mutations distributions. It therefore describes how the evolution of the population selects for some particular arrangement of correlated allelic values for the various traits. Indeed, the association of allelic values for the different traits influence the number of combinations to produce given values of traits, and thus influences the conjoint transmission of traits and the variance of genotypes in the population. This is demonstrated by the results showing that these bootstrap values converge - depending on the simulation scenario - towards specific values, this pointing at a particular organisation of correlated allelic values with respect to the spatial structure of the genome (Figure 8). Additionally, the model also calculates such measure at the individual level to observe how the correlation of allelic effect is related to fitness (Figure B3, supplementary material).
We hope to have clarified our approach and its interest, and we would welcome your feedback. We believe this exchange will be valuable to us in resubmitting an improved version of the paper to PCI.
Sincerely Yours,
Louise Chevalier and Jacques Labonne.
Decision by Michael D Greenfield, posted 11 Jun 2020
It is clear that this manuscript addresses an important issue in evolutionary biology and animal behavior, and one that could benefit from more modeling and simulation. However, it is also clear that the overall objectives and modeling approach of the paper need to be clarified, that an assessment of the validity of the model should be attempted, and that many genetic details need correction. Reviewer 1 has called attention to the lack of reference to other modeling approaches currently in wide use and how the manuscript's method compares with them. Reviewer 2 offers detailed suggestions for overall improvement and for sharpening the presentation of the study. All of these points will have to be addressed before a recommendation can be considered.
Michael Greenfield.
Additional requirements of the managing board:
As indicated in the 'How does it work?’ section and in the code of conduct, please make sure that:
-Data are available to readers, either in the text or through an open data repository such as Zenodo (free), Dryad or some other institutional repository. Data must be reusable, thus metadata or accompanying text must carefully describe the data.
-Details on quantitative analyses (e.g., data treatment and statistical scripts in R, bioinformatic pipeline scripts, etc.) and details concerning simulations (scripts, codes) are available to readers in the text, as appendices, or through an open data repository, such as Zenodo, Dryad or some other institutional repository. The scripts or codes must be carefully described so that they can be reused.
-Details on experimental procedures are available to readers in the text or as appendices.
-Authors have no financial conflict of interest relating to the article. The article must contain a "Conflict of interest disclosure" paragraph before the reference section containing this sentence: "The authors of this preprint declare that they have no financial conflict of interest with the content of this article." If appropriate, this disclosure may be completed by a sentence indicating that some of the authors are PCI recommenders: “XXX is one of the PCI XXX recommenders.”
Reviewed by anonymous reviewer 1, 16 May 2020
The paper presents itself as a proof-of-principle for “demogenetic agent-based models” in evolution. I am having difficulty distinguishing their approach from individual-based simulations that are widespread in the literature, like SLiM, that are somehow not cited here. I feel like they use a vocabulary that creates unnecessary distinctions and confusion with respect to most of the literature on population genomics. I am still wrapping my head around the notion of a “mean genome” The paper does not engage contemporary simulation-based work or population-genomic theory. I think it needs to be rewritten extensively to clarify the distinctions with other agent-based models and the unique contributions this approach could make, if any.
https://doi.org/10.24072/pci.evolbiol.100236.rev11Reviewed by Frédéric Guillaume
, 08 Jun 2020
I have now reviewed the manuscript "A demogenetic agent based model for the evolution of traits and genome architecture under sexual selection" By Chevalier et al. This work describes a simulation program to model the joint action of mating preference, mating system, and genetic architecture. The intention is to simulate the effects of sexual selection on the evolution of the mating system. Although there are many individual-based, genetically explicit evolutionary simulation software available, I am not aware of any who has implemented such models yet. The model allows the user to address fundamental questions on evolution under sexual selection, a key evolutionary force in nature.
I have a number of concerns about the implementation of the model, and especially about the interpretation of the genetic output. I will mostly comment on the genetic architecture.
Major comments:
As said, it is one among many individual-based, forward-in-time, genetically explicit, stochastic simulation software available "on the market". Yet, the manuscript doesn't reference any of those other tools. The authors should acknowledge that diversity and position their tool relative to what new it brings and how different it is from the rest.
(My feeling is that the simulation software is designed to address specific questions in a specific context with a specific model. The general approach (demogenetic agent-based simulations = eco-evolutionary individual-based simulations) is well developed in other more advanced and flexible simulators. The audience and use of the software is thus likely to remain specific as well. Which is fine of course.)
The manuscript is part a tool description and part a model description. While it is not always easy to clearly separate the two aspects of the approach, the authors should focus on one of the two aspects. If the goal is to propose a new, cool simulation software, then less room should be dedicated to the description and interpretation of the results of the simulations. The manuscript would then greatly gain in readability.
I am missing information about how users can do to use that software, how they can interact with it and run simulations, etc.
If the goal were not to "sell" a simulation software, then the focus should be made on the evolutionary question and less on the simulation approach. For now, it is a bit a hybrid between the two and might miss its audience.
One of my major criticism is that the authors did not care to validate their simulation results with known theoretical results. The results presented are of little value because we do not have a way to assess their validity. What assures us that the simulator is capable of producing reasonable outcomes based on known expectations?
The rest of my comments are relative to the genetic setting and interpretation of the simulations.
>>Polygenicity:*
LL318-323: I don't agree with the definition of the "degree of polygeny" as the number of loci of major effects. If anything, the degree of polygeny should DECREASE with the number of loci of major effects. The higher the number of loci affecting (equally) a trait, the higher the polygenicity. A polygenic trait is defined as a trait affected by many loci of small effect. What you describe is a degree of "oligo-genicity". Please better justify your choice here.
>>Pleiotropy:*
LL243-254: I don't agree with the interpretation of pleiotropic and non-pleiotropic allele values here. Allele "0" is not equivalent to "neutral" because phenotypic value "0" can be selected for. The optimum trait value can be 0 (it can also be negative). Quantitative phenotypes and alleles have in principle unbounded continuous values. Thus, modularity cannot be inferred from the allelic distribution. Instead, modularity of the genotype-phenotype map is typically evaluated from the pattern of genetic covariance between traits. Traits are said independent when they are not correlated, their covariance is zero. Trait modularity is found when traits can be grouped within modules of correlated traits. However, modularity at the gene level is a function of the pleiotropic connections of the genes to the traits and here, unfortunately, the "0" allele doesn't count as an absence of pleiotropic connection.
LL324-337: What you describe here is not what is commonly understood as the "degree of pleiotropy", but is closer to the correlation of the allelic effects. It is also unnecessarily complicated. You should clarify and justify why you use a different approach in your case. If/when genes have similar allelic values on different traits, those traits will become genetically correlated. Traditionally, this is investigated by measuring the genetic variance-covariance matrix (the G-matrix) of the traits. The genetic independence of the traits is then evaluated from the structure of the G-matrix, especially from its modularity. See discussion in Chebib, J. & Guillaume, F. (2017) What affects the predictability of evolutionary constraints using a G-matrix? The relative effects of modular pleiotropy and mutational correlation. Evolution, 71:2298-2312. Arnold, S. J.; Bürger, R.; Hohenlohe, P. A.; Ajie, B. C. & Jones, A. G. (2008) Understanding the evolution and stability of the G-matrix. Evolution, 62:2451-2461
Note that absence of genetic correlation is not synonymous of absence of pleiotropy because genes can be fully pleiotropic (affect all traits) and yet, the traits may remain uncorrelated genetically and phenotypically (like you observe in your simulations). The pleiotropic degree (the number of traits affected by a gene) is thus not informative of the correlation among traits. If you intend to keep using the index of "pleiotropic degree", you should rename it to avoid confusion with the more common use of this term.
L409: this not an absence of pleiotropy but an absence of correlation among allelic effects. Pleiotropy does not evolve in the model, each locus is still affecting each trait. You are confounding genetic correlation with pleiotropy. Here, the correlation among allele values at the pleiotropic loci is apparently under selection.
L417: referring to comments above, the emerging modular genetic architecture is caused by the evolution of the genetic covariance among traits, and not of pleiotropy itself.
LL582-584: it is not a new or surprising result that genetic correlation can increase without a change in pleiotropy. All is needed is an increase in the co-variation of the genotypic values of the corresponding traits. The way the allelic values are distributed within genes will not necessarily reflect on the trait correlation as long as it varies among genes, because what matters is the sum of those effects on the traits. I would thus not call pleiotropy the difference in allele values at a particular locus as it is anyway strongly dependent on mutational input, moreover when using a strongly skewed mutational effect distribution. Therefore, the pattern shown in Fig 5 is not likely to be very stable over time and likely to be idiosyncratic.
>>Mutational effect distribution:
L136: why a Beta distribution? justify
L237: what justifies your choice of Beta distribution for allelic values at your quantitative trait loci? The mutational distribution of quantitative trait loci (QTL) is typically assumed a Gaussian (multivariate Normal) and not Beta in the literature on quantitative genetics. Are you referring to the distribution of deleterious mutations? This shouldn't apply to QTL.
LL241-242, Fig 3: it is not possible to evaluate the validity of your genetic parameters and assumptions since no theoretical expectation exists for the Beta distribution of mutational effects. The expected variance of a quantitative trait determined by additive loci is given by the stochastic house-of-cards approximation at mutation-selection-drift balance under the assumption of normally distributed mutational effect with variance alpha^2 and mean 0: Vg = (2 NeVm)/(1 + (Nealpha^2/Vs)), with mutational variance Vm = 2Lmu*alpha^2 (L=number of loci, mu=mutation rate), strength of (Gaussian) selection: Vs = omega^2+Vp. See references in Turelli, M. (1984). Heritable genetic variation via mutation-selection balance: Lerch’s zeta meets the abdominal bristle.Theor.Popul.Biol., 25(2):138–193; Burger, R. (2000). The mathematical theory of selection, recombination, and mutation. In Levin,S., editor, Wiley series in Mathematical and Computational Biology. Wiley & Sons, Ltd, UK
LL498-501, Figs 9-10: the difference of the evolutionary dynamics between the 10loci and 100loci architectures seems suspicious to me. Did you check that the mutational variance per trait is the same in both models? the evolutionary dynamics should be the same for a similar level of mutational input to the variance (Va) and covariance of the traits. Because you use a non conventional mutational distribution for quantitative loci, it is very difficult to evaluate if biases were introduced by your modelling choice. I surmise that the difference between the 10L and 100L cases is caused by differences in the mutational variance-covariance of the traits. You should compare your results with simulations using a multivariate Normal distribution for the mutational effects.
Mating groups:* LL157-158: explain why and how should the user decide to divide the population into mating groups. What is the rational behind this modelling choice? How should the user choose a value for M?
L162: random mating: this procedure describes a monogamous mating system and not what is more commonly understood as "random mating" where all gametes from all individuals mix freely (also taking account of gender). The difference would be that, under random mating, the offspring of a particular female are not all from the same male, in contrast to monogamy. Here, you need to clarify how many offspring are created from each mating and whether your mating system differs from monogamous mating.
Minor comments
L13: polygyny -> polygenicity
L18: retroactions -> feedback
L105: stick to one term, either agent-based or individual-based
L121: The process for simulations -> The simulation process
L123: "procedures are processed" -> better call those procedures something like "life cycle events", "procedure" is more of a technical word
L125: probability of surviving for individuals -> the "individual surviving probability" or "surviving probability of individuals"
LL126,144: what are "costly traits"? clarify how these costs are expressed and set
L128: drawing "lots" of individuals -> be more specific, what and how much is "lots"
L131: replace comma with dot, start a new sentence at "Their sex..."
LL 134-135: not clear if "New alleles are assumed to be created by mutation" means something different from the statement in previous sentence. Sounds redundant.
L148: in considering -> when considering
L149: remove "individual" ? meaning unclear
LL171-172: what stops that iterative procedure?
L176: italicize "M" throughout the manuscript
LL188-189: what is meant here is not clear. What stops the sequential procedure? how many matings are processed and how is a non-effective mating affecting the subsequent ones?
L191: an individual is not a "he": "his" -> "its"
L223: per loci -> per locus
LL224-225: more recent citations on mutation rates should be given. Progresses have been made in estimating mutation rates from genomic data during the last decade.
L230: Alleles effects -> Allelic effects
LL251,253: use gender neutrality for "individual": "he" -> "it" (or feminise them, for a change)
LL273-280: make extra clear that the costs mentioned are all implicit, no parameter allows the user to set those costs explicitly
L284: the purpose of these comments isn't clear, are they necessary?
L287: not clear what you mean with "relative to other strategies". It should be strategies of the other individuals
L292: first time the extrinsic environment is explicitly mentioned but without details. How is that environment set? specify
L294: gender neutrality!
L366: what conditions are necessary for a pseudo-equilibirum versus an equilibirum? the distinction should be clarified for the rest of the examples.
L416: not clear what a mutational landscape is. You should refer to the distribution of mutational effect for clarity.
https://doi.org/10.24072/pci.evolbiol.100236.rev12