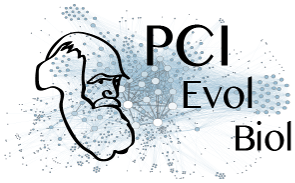
Inference of genome-wide processes using temporal population genomic data
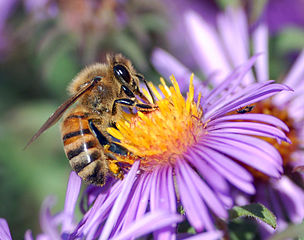
Joint inference of adaptive and demographic history from temporal population genomic data
Abstract
Recommendation: posted 21 November 2022, validated 29 November 2022
Tellier, A. (2022) Inference of genome-wide processes using temporal population genomic data. Peer Community in Evolutionary Biology, 100158. https://doi.org/10.24072/pci.evolbiol.100158
Recommendation
Evolutionary genomics, and population genetics in particular, aim to decipher the respective influence of neutral and selective forces shaping genetic polymorphism in a species/population. This is a much-needed requirement before scanning genome data for footprints of species adaptation to their biotic and abiotic environment (Johri et al. 2022). In general, we would like to quantify the proportion of the genome evolving neutrally and under selective (positive, balancing and negative) pressures (Kern and Hahn 2018, Johri et al. 2021). We thus need to understand patterns of linked selection along the genome, that is how the distribution of genetic polymorphisms is shaped by selected sites and the recombination landscape. The present contribution by Pavinato et al. (2022) provides an additional method in the population genomics toolbox to quantify the extent of linked positive and negative selection using temporal data.
The availability of genomics data for model and non-model species has led to improvement of the modeling framework for demography and selection (Johri et al. 2022), but also new inference methods making use of the full genome data based on the Sequential Markovian Coalescent (SMC, Li and Durbin 2011), Approximate Bayesian Computation (ABC, Jay et al. 2019), ABC and machine learning (Pudlo et al. 2016, Raynal et al. 2019) or Deep Learning (Sanchez et al. 2021). These methods are based on one sample in time and the use of the coalescent theory to reconstruct the past (demographic) history. However, it is also possible to obtain for many species temporal data sampled over several time points. For species with short generation time (in experimental evolution or monitored populations), one can sample a population every couple of generations as exemplified with Drosophila melanogaster (Bergland et al. 2010). For species with longer generation times that cannot be easily regularly sampled in time, it becomes possible to sequence available specimens from museums (e.g. Cridland et al. 2018) or ancient DNA samples. Methods using temporal data are based on the classical population genomics assumption that demography (migration, population subdivision, population size changes) leaves a genome-wide signal, while selection leaves a localized signal in the close vicinity of the causal mutation. Several methods do assess the demography of a population (change in effective population size, Ne, in time) using temporal data (e.g. Jorde and Ryman 2007) which can be used to calibrate the detection of loci under strong positive selection (Foll et al. 2014). Recently Buffalo and Coop (2020) used genome-wide covariance between allele frequency changes across time samples (and across replicates) to quantify the effects of linked selection over short timescales.
In the present contribution, Pavinato et al. (2022) make use of temporal data to draw the joint estimation of demographic and selective parameters using a simulation-based method (ABC-Random Forests). This study by Pavinato et al. (2022) builds a framework allowing to infer the census size of the population in time (N) separately from the effect of genetic drift, which is determined by change in effective population size (Ne) in time, as well estimates of genome-wide parameters of selection. In a nutshell, the authors use a forward simulator and summarize genome data by genomic windows using classic statistics (nucleotide diversity, Tajima’s D, FST, heterozygosity) between time samples and for each sample. They specifically use the distributions (higher moments) of these statistics among all windows. The authors combine as input for the ABC-RF, vectors of summary statistics, model parameters and five latent variables: Ne, the ratio Ne/N, the number of beneficial mutations under strong selection, the average selection coefficient of strongly selected mutations, and the average substitution load. Indeed, the authors are interested in three different types of selection components: 1) the adaptive potential of a population which is estimated as the population mutation rate of beneficial mutations (θb), 2) the number of mutations under strong selection (irrespective of whether they reached fixation or not), and 3) the overall population fitness which is a function of the genetic load. In other words, the novelty of this method is not to focus on the detection of loci under selection, but to infer key parameters/distributions summarizing the genome-wide signal of demography and (positive and negative) selection. As a proof of principle, the authors then apply their method to a dataset of feral populations of honey bees (Apis mellifera) collected in California across many years and recovered from Museum samples (Cridland et al. 2018). The approach yields estimates of Ne which are on the same order of magnitude of previous estimates in hymenopterans, and the authors discuss why the different populations show various values of Ne and N which can be explained by different history of admixture with wild but also domesticated lineages of bees.
This study focuses on quantifying the genome-wide joint footprints of demography, and strong positive and negative selection to determine which proportion of the genome evolves neutrally or not. Further application of this method can be anticipated, for example, to study species with ecological and life-history traits which generate discrepancies between census size and Ne, for example for plants with selfing or seed banking (Sellinger et al. 2020), and for which the genome-wide effect of linked selection is not fully understood.
References
Johri P, Aquadro CF, Beaumont M, Charlesworth B, Excoffier L, Eyre-Walker A, Keightley PD, Lynch M, McVean G, Payseur BA, Pfeifer SP, Stephan W, Jensen JD (2022) Recommendations for improving statistical inference in population genomics. PLOS Biology, 20, e3001669. https://doi.org/10.1371/journal.pbio.3001669
Kern AD, Hahn MW (2018) The Neutral Theory in Light of Natural Selection. Molecular Biology and Evolution, 35, 1366–1371. https://doi.org/10.1093/molbev/msy092
Johri P, Riall K, Becher H, Excoffier L, Charlesworth B, Jensen JD (2021) The Impact of Purifying and Background Selection on the Inference of Population History: Problems and Prospects. Molecular Biology and Evolution, 38, 2986–3003. https://doi.org/10.1093/molbev/msab050
Pavinato VAC, Mita SD, Marin J-M, Navascués M de (2022) Joint inference of adaptive and demographic history from temporal population genomic data. bioRxiv, 2021.03.12.435133, ver. 6 peer-reviewed and recommended by Peer Community in Evolutionary Biology. https://doi.org/10.1101/2021.03.12.435133
Li H, Durbin R (2011) Inference of human population history from individual whole-genome sequences. Nature, 475, 493–496. https://doi.org/10.1038/nature10231
Jay F, Boitard S, Austerlitz F (2019) An ABC Method for Whole-Genome Sequence Data: Inferring Paleolithic and Neolithic Human Expansions. Molecular Biology and Evolution, 36, 1565–1579. https://doi.org/10.1093/molbev/msz038
Pudlo P, Marin J-M, Estoup A, Cornuet J-M, Gautier M, Robert CP (2016) Reliable ABC model choice via random forests. Bioinformatics, 32, 859–866. https://doi.org/10.1093/bioinformatics/btv684
Raynal L, Marin J-M, Pudlo P, Ribatet M, Robert CP, Estoup A (2019) ABC random forests for Bayesian parameter inference. Bioinformatics, 35, 1720–1728. https://doi.org/10.1093/bioinformatics/bty867
Sanchez T, Cury J, Charpiat G, Jay F (2021) Deep learning for population size history inference: Design, comparison and combination with approximate Bayesian computation. Molecular Ecology Resources, 21, 2645–2660. https://doi.org/10.1111/1755-0998.13224
Bergland AO, Behrman EL, O’Brien KR, Schmidt PS, Petrov DA (2014) Genomic Evidence of Rapid and Stable Adaptive Oscillations over Seasonal Time Scales in Drosophila. PLOS Genetics, 10, e1004775. https://doi.org/10.1371/journal.pgen.1004775
Cridland JM, Ramirez SR, Dean CA, Sciligo A, Tsutsui ND (2018) Genome Sequencing of Museum Specimens Reveals Rapid Changes in the Genetic Composition of Honey Bees in California. Genome Biology and Evolution, 10, 458–472. https://doi.org/10.1093/gbe/evy007
Jorde PE, Ryman N (2007) Unbiased Estimator for Genetic Drift and Effective Population Size. Genetics, 177, 927–935. https://doi.org/10.1534/genetics.107.075481
Foll M, Shim H, Jensen JD (2015) WFABC: a Wright–Fisher ABC-based approach for inferring effective population sizes and selection coefficients from time-sampled data. Molecular Ecology Resources, 15, 87–98. https://doi.org/10.1111/1755-0998.12280
Buffalo V, Coop G (2020) Estimating the genome-wide contribution of selection to temporal allele frequency change. Proceedings of the National Academy of Sciences, 117, 20672–20680. https://doi.org/10.1073/pnas.1919039117
Sellinger TPP, Awad DA, Moest M, Tellier A (2020) Inference of past demography, dormancy and self-fertilization rates from whole genome sequence data. PLOS Genetics, 16, e1008698. https://doi.org/10.1371/journal.pgen.1008698
The recommender in charge of the evaluation of the article and the reviewers declared that they have no conflict of interest (as defined in the code of conduct of PCI) with the authors or with the content of the article. The authors declared that they comply with the PCI rule of having no financial conflicts of interest in relation to the content of the article.
Evaluation round #2
DOI or URL of the preprint: https://doi.org/10.1101/2021.03.12.435133
Version of the preprint: 4
Author's Reply, 09 Nov 2022
Decision by Aurelien Tellier
, posted 10 Nov 2022, validated 17 Oct 2022
Dear authors,
The three reviewers and myseld acknowledge the efforts you have made in preparing this revision which addresses satisfactorily most critical points and comments. The reviewers have few last minor comments for you to incorporate before I can proceed with the recommendation. I anticipate that this can be done relatively quickly. I anticipate to evaluate your reply to these comments myself.
Best regards and sorry for the delay in obtaining all reviews.
Aurelien Tellier
Reviewed by anonymous reviewer 1, 14 Sep 2022
Summary of the manuscript:
The authors developp an ABC-random forest approach to simultaneously infer census population size (N), effective population size (Ne) and the mutation rate of benefical mutations (theta_b) from temporal population genomic data. To do so they simulate genomic data under selection using Slim to train their ABC-rf and then demonstrate the accuracy of their approach on simulated data in different cases. They then apply their approach on genomic data of different bee population which were collected at different time points. The authors find effective population size very similar to census size and further discuss this result.
General comments :
In this version of the manuscript, I found the material and method section clearer. Some pointed limits/issues were not addressed but I align with the editor's decision. I understand the authors could not addressed all comments as it would be an unreasonable amount of work and go beyond the scope of this study. Hence, I'm happy the authors mentioned that they will try to address some of the comments in a future project/manuscript, which I am looking forward to read.
Minor comments :
-l 308-309, Please add a link here to the VCF files used in this study (e.g. https://github.com/mnavascues/ABC_TimeAdapt/tree/master/data). I am sorry if I overlooked how to access to the data, and authors can ignore my comment. Yet, I looked in the manuscript authors have referred to, but could also not find the mentionned VCF files (I could only find how to access the raw reads). If authors downloaded the data, I would appreciate if the link was written in the manuscript. If the authors were given the data, I would appreciate if they would make the VCF files accessible for everyone. By sharing the data, other people can try their method on a dataset, participating the diffusion of their work and of science. I believe sharing the VCF files would fit with the spirit and the recommandation guidelines of PCI. I am also okay if the files are shared after publication of the paper and/or acceptance in PCi (but it would be nice to state it clearly).
Reviewed by Lawrence Uricchio, 07 Oct 2022
I appreciate the author's responses to the first round of reviews. I do think the manuscript is improved, and remains quite interesting.
I have two remaining comments, which correspond to aspects of their reply. They are really quite minor, although they take some space to explain.
1. The spirit of my comment about including some additional discussion of Poisson Random Field and MK-based work is because many readers will naturally compare this approach to PRF, which is still very widely used for inferring similar models. I agree that PRF is less likely to be useful for such short time periods (~10 generations), and that it doesn't account for linked selection. But it can infer genome-wide selection and demographic parameters. Many readers looking at your Figure 1 will wonder specifcally how/when your method improves on PRF since it solves a model that is (conceptually) identical to models that were solved with PRF (e.g. Boyko et al 2008). Under which scenarios should we prefer an approch like yours to PRF? Highliting the deficiencies of SFS-based analyses for short timescale changes in population size and stating when specifically PRF-based approaches will fail due to linkage would be helpful. How many generations is too few to infer an expansion (as in your model) with PRF? How much linked selection is needed before the problem is severe with PRF? Even a couple of sentences would be helpful.
2. The authors write in their reply "Therefore, we are more interested in the short term effects of negative selection on allele frequency changes than in long term effects on the site frequency spectrum." I think the issue here is that the allele frequency dynamics themselves are not independent of the population history in burn-in. With low recombination and a high rate of beneficial alleles, the dynamics may look more like clonal interference. If there is a lower rate of positive selection but high backgriound selection, the trajectories of the beneficial alleles may be subject to less perturbation by interference than an equivalent reduction in Ne due to positive selection, but the effect depends on the selection coefficients. I appreciate the section on limitations that discusses background selection, but it is not very clear in the manuscript how this will affect inference under this model. Some discussion of the ways that negative seleciton might affect the parameter inference would be helpful.
Reviewed by anonymous reviewer 2, 13 Oct 2022
The authors have mostly addressed my concerns. However, I was taken aback by the reluctance to estimate the robustness to deleterious mutations given how easy it is to simulate nowadays. It would have been straightforward to do. In their defense, the authors have added discussion about this potential limitation, in particular suggesting that heterogenous background selection and deleterious mutations could be problematic. The approach has potential, so I do hope that the authors explore these questions in the future to validate the goals behind their approach.
Evaluation round #1
DOI or URL of the preprint: https://doi.org/10.1101/2021.03.12.435133
Version of the preprint: 2
Author's Reply, 07 Sep 2022
Decision by Aurelien Tellier
, posted 17 Jan 2022
Dear authors,
I am sorry for the delay in the evaluation of your manuscript. I had trouble finding reviewers at first, but then found three very enthusiastic reviewers, but the end of the year holidays delayed their reviews. Your manuscript is very interesting and I hope you will consider submitting a revised version.
You will find that all reviewers are very enthusiastic about the manuscript and very supportive (see attached reviews). The reviewers evaluated thoroughly your manuscript and suggest a number of minor changes to improve clarity of the methods and results. These should be easily doable.
In addition, two reviewers suggest potential additional simulations to test for the effect of heterogeneous recombination, heterogeneity of deleterious mutations in genes and demography on the accuracy of your estimations. While I am aware that it would require a substantial amount of work to do all these tests, I would recommend to test by simulations the effect of heterogeneous recombination on your method accuracy. The other potential biases can be dealt with in the discussion (you will find also some useful suggestions to improve the discussion and add some additional references).
I look forward to receive your revised version and will make sure the review process get faster in the next round,
Many thanks for submitting to PCI,
Aurelien Tellier
Reviewed by anonymous reviewer 1, 08 Dec 2021
Summary of the manuscript:
The authors developp an ABC-random forest approach to simultaneously infer census population size (N), effective population size (Ne) and the mutation rate of benefical mutations (theta_b) from temporal population genomic data. To do so they simulate genomic data under selection using Slim to train their ABC-rf and then demonstrate the accuracy of their approach on simulated data. They then apply their approach on genomic data of different bee population which were collected at different time points. The authors find effective population size very similar to census size and further discuss this result.
General comments :
I found the manuscript interesting and I enjoyed reading it. The authors try to answer a question which is central in the field of population genetics and of conservation biology. However I believe the manuscript can be inmproved by addressing two points. First, I think the Method section is a little bit confusing (see below for further details) and the Material is not well described. Secondly, depending on the recommender's decision, I think few additional analyses could complete their study.
Major comments :
- I find the ABC-rf to be well defined but not the data on which it was applied. In the main text (or in the appendix) I could not find basic information on the input data such as sample size of simulations or of the data (or if sample size at t1 was equal to sample size at t2, which does not seem to be the case according to what is written l 442). I believe the authors should add a table (in appendix or main text) describing what they simulated and the data they have used. Currently, the input data is very cryptic and I'm not sure results could be reproduced from the main text only.
With the recommender's approaval I would also address those two points :
- I think one or two supplementary analyses are missing. The Authors assume census population size to be constant in presence of selection (if I understood correctly). I think it's necessary to run their ABC-rf on data that has been simulated with non-constant population size (e.g. bottleneck) but under neutrality. Secondly, and they mention it in the discussion, it would be interesting if they could run their approach on data simulated under neutrality, constant population size but in presence of migration. I believe those two analyses could help the authors to better understand the performance of ABC-rf on their data and strengthen their discussion.
- The Authors mention summary statistics being used by the random forest to perform the inference, yet no summary statistic of the data are displayed in the manuscript or in the Supplementary files. As a sanity check I would add few Supplementary Figures/Tables displaying the "interesting"/"Selected" summary statistics of the data (based on S4 and S5), and the same summary statistics calculated from the simulations where parameters are set to the most likely (i.e. with highest density) one (Figure 4). If summary statistic calculated from data are similar than those of the simulated data, it would support their conclusions. On the opposite, divergence between both could indicate an underlying non-accounted process, which they also mention in the discussion.
Minor comments :
Abstract:
-l 24, what do you mean by "realized potential" ?, I would rephrase
Introduction
-l 50, I would define "type I error rate" in the text for clarity
-l 80-95, you mention your methods to be accurate and that random forest has solved the computation issue, yet you didn't presented your input data. It would be usefull to have a sentence/paragraph describing the input data.
Methods
-l 107, if you assume a constant population size of N, please mention it more clearly here.
-l 126, I think you should also add the sample size here.
-l 127-128, from the text it seems like you sampled individuals at time t1 and t2, with tau = t2-t1. But from Figure 1 A), it seems like you have been sampling between t1 and t2. In addition, tau is missing on the Figure 1. I would change Figure 1 A) by remplacing "sampling period" by "tau".
-l 133, I would change "c0" to "r0" for the recombination rate as I think it's a more classic notation.
-l 225-249, I do not think all variables have been clearly defined in the previous sections and there might be some inconsistent notations, e.g. is r="c0" ? I think it would help readers if the authors moved Table S1 into the main text (but r is missing from table S1).
-l 285, Once the paper is accepted/recommended please add a link here in the text to the individual VCF files.
-Table 1, what is written in column N (and add it to the table description) ?
-l 290-309, I didn't find where the sample size you are using was written. Could you add it in the text or in Table 1 ?
Results :
-Figure 4, I think there is a typo in the legend, c) and a) should be exchanged.
-Figure 4, I think having a small table summarizing Figure 4 with the most likely (or whith highest density) ratio of Ne/N would help in understanding the results.
Discussion :
-l 440-498, The authors discuss the similarity between Ne and N and they mention the possibility of excluding lower values of theta_b indicating acting selection during the study (by giving explanation on why signature of selection might be harder to find). Could it really not be neutral ?
- I don't know if authors have read the recent paper of Barroso et al (https://doi.org/10.1101/2021.09.16.460667) on the inference of the mutation rate map from whole genome data. In their study they interpret the variation of the Ne along the genome as a the variation of the mutation rate. However, if you assume the mutation to be constant along the genome, then they would infer the variation of Ne along the genome. I wonder if the inferred mutation rate map would be an interesting summary statistic for you ABC-rf ? I would recommand the authors to read the study as it could be relevant in the light of their work (and maybe add it to the discussion).
Reviewed by anonymous reviewer 2, 14 Jan 2022
In their preprint, Pavinato et al. describe an ABC Random Forest approach to jointly infer demography and selection in population genomics, two-time points temporal data. Overall I like the approach a lot, especially the fact that it can be extended to more realistic situations that for example include deleterious mutations. Reading the preprint I had an issue with semantics, that has to do with the fact that the authors do not really infer demography per se, but rather provide an approach that allows to estimate selection while also jointly estimating the influence of “whatever” past demography occurred. This is different from what most people in the field understand by “inferring demography”, which usually means explicitly inferring population size changes over time. This needs to be made clearer throughout the manuscript.
I have no concern with the overall approach that does sound very promising. However, I have two main concerns regarding the practical usability when faced with actual datasets that will likely present 1) recombination rate heterogeneity and 2) varying levels of deleterious mutations across loci.
1) Recombination. The authors use uniform recombination in their approach. We know however that many genomes have highly heterogeneous distributions of recombination events, and those even often co-vary with with functional elements in genomes. For example in human genomes, recombination hotspots tend to co-coccur together with regulatory elements. This is an example where not accounting for this heterogeneity is a big issue given that any approach with uniform recombination will then likely underestimate adaptation that occurs in these functional elements. The authors need to test with simulations with heterogeneous recombination how much their approach is affected, especially when the recombination hostpots tend to occur near where the adaptation is expected in the first place.
2) Deleterious mutations. In the same spirit, instead of just discussing very briefly about this limitation, the authors could actually run a few simulation to see how their approach performs when the test, simulated genomes actually include deleterious mutations. What happens when the deleterious mutations are distributed evenly across loci, and what happens when the deleterious mutations are heterogeneously distributed across loci. The deviations from the expected values especially for adaptation should then provide more solid ground to discuss the limitations in much more detail than it is done at the moment.
I am suggesting thes two things (recombination and deleterious mutations) since it would not take too many additional simulations with SLIM to get a more precise sense of how not accounting for them biases the estimates.
In addition I just have some minor comments about a few things that need to be better defined in the Introduction. First, the authors need to better describe how Random Forest works and why this works better in the context of ABC. Second, the authors need to better define early in the introduction what latent variables are, how they work, and what their usefulness is in the approach.
Reviewed by Lawrence Uricchio, 12 Jan 2022
"Joint inference of adaptive and demographic history from temporal population genomic data" by Pavinato et al explores the inference of demographic parameters and selection parameters using random forests and Approximate Bayesian Computation. They present an analysis of their simulation-based inference procedure as well as an application of their method to genomic time-series data from honey bees. The manuscript addresses an interesting and important problem, and is overall clear and well-written. I very much appreciated the thorough investigation of the performance of their method. I have some questions and suggestions for the authors. I want to emphasize that although I have several comments, I do very much appreciate the authors' work and found the manuscript quite interesting.
Main comments:
1. Overall I found the descriptions of the analyses to be clear, but how they fit with the existing literature on joint inference of selection and demography was sometimes less clear. Some important areas of the literature on joint selection/demography inference are not mentioned in the manuscript (described more in the next point). It would be very helpful if the authors could further clarify how the approach that they take (random forests with ABC) provides an advance over the range of previous approaches. Their method aims to directly account for the effects of linked selection (which I agree is an important goal), but whether their method actually performs better in a practical sense than methods that do not explicitly model linked selection does not seem to be assessed. The effect of the random forests on the performance relative to ABC without the inclusion of the random forests is also not mentioned, although this may be a less feasible comparison given computational efficiency limitations.
2. The authors state in the introduction that "Methods for demographic inference assume that most of the genome evolves without the influence of selection and that any deviation from the mutation-drift equilibrium observed in the data was caused by demographic events". I agree in the sense that the effects of linked selection are often ignored in such analyses, but there are many studies that attempt to disentangle selection/demography by dividing the genome into putatively selected sites and putatively neutral sites.
Such methods are derived from the PRF (Poisson Random Field) and/or MK (McDonald-Kreitman) framework and assume that demographic events shape patterns of variation at putatively neutral sites (often taken as synonymous alleles within genes) while both selection and demography shape putatively selected sites (sometimes taken as nonsynonymous alleles within genes). Patterns of variation at the "neutral" sites are used to fit the demographic model while the "selected" sites are used to fit the selection model. There are many such papers (e.g. one could start with Williamson et al 2005 PNAS and Keightley & Eyre-Walker 2007 Genetics, or a more recent paper such as Racimo & Schraiber 2014 Plos Genetics).
The realization that putatively neutral sites may not be sufficiently 'neutral' for such analyses (due to direct or indirect selection) has also been a long-standing topic of discussion, and some studies have sought to address this issue. For example, Gazave et al 2014 (PNAS) sequenced regions far from genes in humans to try to fit demographic models that were less affected by selection. Torres, Szpiech, & Hernandez 2018 (Plos genetics) found that background selection has a substantial influence on demographic inference in humans. Messer & Petrov 2013 (PNAS) developed a method to infer adaptation rate and tested its robustness to linked selection and non-equilibrium demography. There are is also at least one paper that seeks to infer recurrent selection and demography using a combination of ABC and PRF methods (N. Singh et al 2013 Genetics, "Inferences of demography and selection...")
The effects of non-equilibrium demography on selection inferences using genetic time series data have also been studied (E.g., Schraiber et al 2016 (Genetics) uses an MCMC-based procedure and compares equilibrium/non-equilibrium demography).
This is just a subset of the papers in this area, and it's not my intent to be prescriptive about citing particular papers. Overall, it would be very helpful for the reader if the authors could put their method into context with this body of work.
3. The authors focus on a model of beneficial alleles and neutral alleles. I found this a bit surprising, given that negatively selected alleles are likely to represent a much larger fraction of mutations and have a substantial effect on the frequency spectrum (and other summary statistics).
The authors discuss the impact of background selection briefly in the discussion, but I think further justification for the relevance of this beneficial allele model (or simulation of a model that includes deleterious alleles, or an argument as to why negative selection will have limited impact on the authors' summary stats) would be helpful here. It is true that some recent papers (e.g. Schrider, Shanku & Kern 2016 Genetics) have focused on positive selection models, but their purpose was to describe the effects of positive selection on demographic inference directly, rather than develop inference procedures. It seems unlikely to me that a model of beneficial alleles alone can provide useful inferences on real data that are certainly affected by a mixture of beneficial alleles and deleterious alleles. Alternatively, if there are specific insights that a beneficial allele model can provide then it would help to further highlight these. I do appreciate that the authors were clear about this limitation in their discussion section.
Minor comments:
-Line 71: Is the '10,000' here years? Or generations?
-Line 80: Another paper that may be of interest here is A. Stern et al Plos Genetics (2019)
-Line 90: AABC is also a way to reduce the number of simulations, see Buzbas & Rosenberg 2015 Theoretical Population Biology.
-Line 120: I'm a bit confused by this description of burn-in. Generally the purpose is to reach the dynamic equilibrium state that is determined by the parameter settings.
-Figure 1: Why are there two different colors for the neutral mutations? Do they represent different things?
-Selection is determined by a Gamma distribution here, which has two parameters. Only the mean of the distribution seems to be mentioned here, which seems to leave one free parameter. How are these parameters selected (perhaps this is mentioned and I missed it)?
-Line 190: I think 'oscillated' may not be the appropriate word here, perhaps fluctuated?
-Line 231: 10 generations seems like a very short time? What is the rationale for this number?
-Line 293: The transition from discussing real to simulated data seems very abrupt here?
I thank the authors and editors for the opportunity to review this very interesting manuscript.
Sincerely,
Lawrence Uricchio