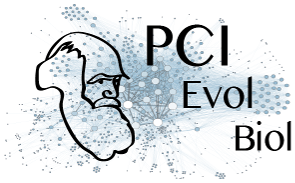
Genetic and environmental robustness are distinct yet correlated evolvable traits in a gene network
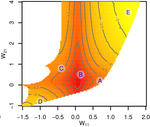
Gene network robustness as a multivariate character
Abstract
Recommendation: posted 08 March 2022, validated 31 March 2022
Guillaume, F. (2022) Genetic and environmental robustness are distinct yet correlated evolvable traits in a gene network. Peer Community in Evolutionary Biology, 100138. https://doi.org/10.24072/pci.evolbiol.100138
Recommendation
Organisms often show robustness to genetic or environmental perturbations. Whether these two components of robustness can evolve separately is the focus of the paper by Le Rouzic [1]. Using theoretical analysis and individual-based computer simulations of a gene regulatory network model, he shows that multiple aspects of robustness can be investigated as a set of pleiotropically linked quantitative traits. While genetically correlated, various robustness components (e.g., mutational, developmental, homeostasis) of gene expression in the regulatory network evolved more or less independently from each other under directional selection. The quantitative approach of Le Rouzic could explain both how unselected robustness components can respond to selection on other components and why various robustness-related features seem to have their own evolutionary history. Moreover, he shows that all components were evolvable, but not all to the same extent. Robustness to environmental disturbances and gene expression stability showed the largest responses while increased robustness to genetic disturbances was slower. Interestingly, all components were positively correlated and remained so after selection for increased or decreased robustness.
This study is an important contribution to the discussion of the evolution of robustness in biological systems. While it has long been recognized that organisms possess the ability to buffer genetic and environmental perturbations to maintain homeostasis (e.g., canalization [2]), the genetic basis and evolutionary routes to robustness and canalization are still not well understood. Models of regulatory gene networks have often been used to address aspects of robustness evolution (e.g., [3]). Le Rouzic [1] used a gene regulatory network model derived from Wagner’s model [4]. The model has as end product the expression level of a set of genes influenced by a set of regulatory elements (e.g., transcription factors). The level and stability of expression are a property of the regulatory interactions in the network.
Le Rouzic made an important contribution to the study of such gene regulation models by using a quantitative genetics approach to the evolution of robustness. He crafted a way to assess the mutational variability and selection response of the components of robustness he was interested in. Le Rouzic’s approach opens avenues to investigate further aspects of gene network evolutionary properties, for instance to understand the evolution of phenotypic plasticity.
Le Rouzic also discusses ways to measure his different robustness components in empirical studies. As the model is about gene expression levels at a set of protein-coding genes influenced by a set of regulatory elements, it naturally points to the possibility of using RNA sequencing to measure the variation of gene expression in know gene networks and assess their robustness. Robustness could then be studied as a multidimensional quantitative trait in an experimental setting.
References
[1] Le Rouzic, A (2022) Gene network robustness as a multivariate character. arXiv: 2101.01564, ver. 5 peer-reviewed and recommended by Peer Community in Evolutionary Biology. https://arxiv.org/abs/2101.01564
[2] Waddington CH (1942) Canalization of Development and the Inheritance of Acquired Characters. Nature, 150, 563–565. https://doi.org/10.1038/150563a0
[3] Draghi J, Whitlock M (2015) Robustness to noise in gene expression evolves despite epistatic constraints in a model of gene networks. Evolution, 69, 2345–2358. https://doi.org/10.1111/evo.12732
[4] Wagner A (1994) Evolution of gene networks by gene duplications: a mathematical model and its implications on genome organization. Proceedings of the National Academy of Sciences, 91, 4387–4391. https://doi.org/10.1073/pnas.91.10.4387
The recommender in charge of the evaluation of the article and the reviewers declared that they have no conflict of interest (as defined in the code of conduct of PCI) with the authors or with the content of the article. The authors declared that they comply with the PCI rule of having no financial conflicts of interest in relation to the content of the article.
No specific funding beyond the CNRS basic support to the author
Reviewed by Charles Rocabert
, 18 Mar 2022
The author provided careful answers to my comments and modified the manuscript accordingly.
I do not have any other comments at this stage.
Evaluation round #2
DOI or URL of the preprint: https://arxiv.org/abs/2101.01564
Version of the preprint: 3
Author's Reply, 28 Feb 2022
Decision by Frédéric Guillaume
, posted 14 Jan 2022
Dear Dr Le Rouzic,
Thank you for submitting a revision of your work on "Gene network robustness as a multivariate character". The two previous reviewers and a newer one were satisfied with the most recent version of your work and with your answers their comments. All of them ask for some corrections in the text and more clarifications, especially of the new figures. I recommend that you address their comments, and mine below before we can continue with the recommendation. A new revision will most likely not go to reviewers again.
I welcome the addition of the details about the genetic correlation of the robustness traits. It shows more precisely how different aspects of robustness may evolve more or less independently from each others. It thus greatly improves the quality of the paper. However, it can be argued that the way the evolutionary constraints have been assessed is far from the way networks may evolve in natural systems in the sense that we hardly expect robustness traits to be under direct selection. Indeed, as stressed at different places in the manuscript, robustness is an emergent property of the topology of the networks, which determine the expression phenotypes under selection. Therefore, as also asked by the third reviewer, I would like to see a more thorough justification for the purpose of this approach (imposed direct selection on robustness traits) to guide the reader and avoid misinterpretations about the nature of selection on robustness you expect to see in nature. My interpretation is that you use direct selection as a device to understand the evolutionary properties of the robustness traits. This should be made clearer in the text. What should also be clarified is whether and when you'd expect direct selection on robustness traits to act in natural populations.
A discussion on that very same topic is thus also awaited, especially in the light of the difference of your model with existing models. It is not yet clear from your manuscript how the genetic constraints among robustness traits bear on the evolution of the genetic networks themselves when populations are challenged by the environment but in absence of such artificial direct selection on the robustness traits. I think this is a legitimate question since it is generally expected that robustness is a trait under second-order selection. Thus, I would like to see a general discussion on that topic to help us better understand the significance of your findings in a broader evolutionary context. Such a discussion is missing at the moment but would greatly improve the impact of your paper.
Minor Comments
(line numbers refer to the latex-diff version)
l58 : hypotheses -> hypothesis
ll122-123, 136-137 : please verify if assumptions about constitutive expression are the same as in Ciliberti et al. 2007. Seems to me that their S(0) is similar to your P_0. Also amend accordingly comments about constitutive expression in your vs other models elsewhere in the text.
ll385-387 : is it 1000 generations or 5000 as in the legend of Figure 4?
Figure 4:
Caption: illstrated -> illustrated;
A legend for the meaning of the gray arrows in the top-right figure representing join selection on the two traits should be given to better link with the text (ie. "bivariate selection" is used in the text) (see Mullon's comment).
The hyphenated line in the predicted-observed evolvability graph gives the wrong impression that the two evolvabilities match, while they don't. A 1-1 line would rightly show that the observed evolvability is larger than its predicted value. This should be stressed in the text as well.
Figure 5:
The direction of change as function of direction of selection is very hard to find, maybe split the graphs to make the patterns more discernible?
ll457-459 : avoid repetition of "cumulative"; specify that the cumulative effect is on the phenotype (=gene expression level); maybe specify that evolution is "gradual"?
l468 : dfferent -> different
ll507-508 : not sure which mathematical model is referred to here
l511 : catch -> capture
Reviewed by Diogo Melo, 15 Dec 2021
P2: refered -> referred
P14 asymetrical -> asymmetrical
P14 dfferent -> different
P15 reductionnist -> reductionist
P15 occuring -> occurring
P15 Althought -> Although
P16 tigh -> tight
Reviewed by Charles Mullon
, 14 Dec 2021
The author has done a great job at adressing all the points previously raised, and I believe that the manuscript has much improved. I also appreciate the careful explanation provided in the response to reviewers (point 3 in particular). I only have minor comments remaining.
p. 7. To ensure clarity, please specify how response to selection R is computed.
p. 9. Top. Here you refer to the "stability" of networks A-E presented in Appendix 6. However nowhere in Appendix 6 is the stability measure (rho_S) given. I am guessing you are referring to a more qualitative view of stability here. This is confusing as in the rest of the manuscript, stability is defined quantiatively.
p.10. I found the phrase "the evolution of correlations was driven by the direction of selection (more or less robustness), and not by the orientation of the selection gradient relative to the main evolvability axis" difficult to connect with Figure 4. Could you please clarify how you come to this conclusion?
Figure 4. This figure is a little difficult to parse. I think the main difficutly I had was to understand the meaning of "the direction of selection". I think once this is defined properly (see above), it would be good to explain in the legend of Figure 4 what the different axes correspond to (perhaps with an example).
Figure 5. There are too many overlaying symbols in this figure. Would it be possible to separate Figure 5 into two or three subfigures (and perhaps moving some to an appendix, e.g. correlated vs antagonistic selection)?
p. 14 "dfferent" -> different.
p. 15. Can you please define what you mean by "network complexity"?
p. 15. "can not" -> cannot.
p. 16. "tigh" -> tight.
Reviewed by Charles Rocabert
, 11 Jan 2022
In this manuscript, the various facets of gene network robustness (environmental and genetic robustness) are treated as quantitative characters, and studied in a quantitative genetics framework.
The author uses a regulation network model based on the Wagner model and numerical simulations to show that four different measures of robustness (two measures of environmental robustness, two measures of mutational robustness, as well as one measure of network stability) are partially correlated. This suggests that, while not independent, gene network robustness characters can evolve towards many directions, as confirmed by numerical simulations where robustness characters are under direct positive or negative selection. An analysis of the M-matrix then confirms that mutational correlations between the robustness characters can facilitate or prevent their evolution, and that this multivariate response to selection is predictable.
By quantifying more systematically the multi-dimensional nature of gene network robustness, this work highlights the ability for a genetic network to acquire various levels of robustness depending on selective pressures and mutational correlations between robustness components. Doing so, it provides the reader with a more synthetic vision of what is robustness, how to quantify it and how it evolves.
I appreciated reading this manuscript which nicely tackles a difficult theoretical question. Methods are clearly presented, which allows for a smooth reading. In particular, the usage of a quantitative genetics framework to describe robustness components is an important contribution.
The manuscript has already been improved by a first round of review. I have a few suggestions and comments that can be easily addressed:
- The purpose of numerical simulations in section 3.3 could be better presented. In my opinion, these simulations are here to demonstrate the evolvability of robustness under a direct "abstract" selection on robustness components. As such, it should be clearly stated that stabilising selection simulations (where no perturbations are introduced except inherited mutations) are some sort of control, and should not be understood as a proof of the ability/inability of robustness to evolve indirectly in a more realistic setting.
On this point, it would have been interesting to run additional numerical simulations to study the indirect evolution of robustness against environmental and/or genetic perturbations, and to confront simulation results to the predictions made. However it is another manuscript in itself, and I certainly do not ask the author to do so.
Minor comments:
---------------
- Introduction: As far as I know, some authors have attempted to quantify robustness in multiple dimensions (see e.g. https://doi.org/10.1371/journal.pone.0000434).
- 1) "In addition, in models where the genotype-phenotype association is arbitrary, [...]": please provide references
- 2.5) "to an element of the W matrix": Is a single weight selected at random in the matrix?
- 2.5) "By default n=6 [...] By default n'=3": Does this parametrization influence the evolution of robustness (compared e.g. to the case n'=6)? Similarly the mutation rate sigma_v^2 is probably an important factor triggering the indirect evolution of mutational robustness. It has been treated by the author in Appendix 7, but I think a few words on the choice of parameter values is needed.
- Table 2: Indicates 5000G. But 10,000G is used after (section 3.3).
- Figure 1: Panel A is not so easy to read with the floating labels. Maybe use small horizontal barplots with a color code?
- 3.1) "is robust to the way random networks are generated": All the random networks are generated in the same way, the generation mode of the networks is not explored here.
Evaluation round #1
DOI or URL of the preprint: https://arxiv.org/abs/2101.01564
Version of the preprint: 2
Author's Reply, 18 Nov 2021
Decision by Frédéric Guillaume
, posted 15 Mar 2021
Thank you for submitting your work for a recommendation at PCI Evol Biol. The research you present highlights very important points regarding the evolvability of robustness, a long standing and fundamental question in evolutionary biology. The responses of two reviewers are attached to my evaluation. You will see that both are positive about your manuscript but ask for a few clarifications and possible expansions, which I summarize below. In light of those comments, and my own as well, I recommend to have a round of revision before recommendation. I would thus encourage you to resubmit a revised version of your manuscript.
- One salient point of the reviewers' comments is about the kind of pleiotropy at work in the gene network and how it affects the genetic correlation between the robustness traits. Is pleiotropy direct (as when one gene regulates multiple targets in the network) or implicit (as in affecting the emergent robustness traits without clear patterns of pleiotropic regulation)? Reviewer two suggests to estimate to mutation co-variance matrix (M-matrix) as a way to understand the genetic basis for the genetic correlation among robustness traits. I think it would be very informative, and innovative, to make the link between network structure and M-matrix structure.
- A similar question from both reviewers is about the estimation of the G-matrix and its evolution. As reviewer one points out, the structure of G depends also on the strength of correlational selection, not only on the underlying genetic correlation arising from the structure of the gene network. Is there a tendency to decrease/increase the genetic correlation b/n robustness traits due to selection? Can the strength of correlational selection be deduced from the evolution of the G-matrix and of the M-matrix? A link with previous evolutionary quantitative genetics is awaited and would anchor the paper in a well known theoretical framework.
- It is not clear how the results of the 2-dim network generalizes to larger network. In particular, the 2-dim network shows large neutrality in regulation network for stable expression phenotype. How does this generalise to larger network?
- In general, little is said about the constitutive levels of expression and their role in the evolution of robustness traits. This is a point that should be clarified.
- I attach the manuscript with some additional comments and grammatical corrections; next time, please submit a manuscript with line numbers to help with minor corrections. (grammatical mistakes are highlighted in orange)
Reviewed by Diogo Melo, 24 Feb 2021
Robustness is often treated as the generic property resistance to perturbations, with little differentiation as to how different perturbations can require different kinds of robustness. This manuscript uses simulations to investigate how direct selection for different types of robustness in gene networks lead to different outcomes. By explicitly defining several kinds of perturbations, the manuscript shows that, while the different measures of robustness are correlated, not all types of robustness are equivalent, and observing robustness to several kinds of perturbations in organisms can potentially indicate different adaptations to separate selection pressures. This observed scenario is contrasted with the possibility that robustness to several kinds of perturbations shares the same underlying basis or that different kinds of robustness are completely different.This is a simple and well-motivated investigation, the manuscript is well written and direct. The explicit description of the many axes of what we may convincingly call robustness is a worthwhile contribution and brings some much-needed precision to the discussion of the evolution of robustness. General comments:The inclusion of a measure of stability is an interesting necessity for there to be a stable state to which the system can return after a perturbation, but I'm not sure it would traditionally be included under robustness to perturbations. Network C in the two-gene network illustrates this, as it is somewhat robust but not stable. In any event, the two-dimensional results illustrate nicely the relative independence of the different kinds of robustness.pg 9, 2nd paragraph: "Selection on all robustness components also lead to an indirect response of all other components, which confirms a general genetic correlation." - Not necessarily due to genetic correlations, selection could also be correlated due to phenotypic correlations.- In the next paragraph and figure 4, it's not clear that the correlations themselves are not evolving, thus partially removing the genetic constraint. It would be interesting to measure the correlations over time and investigate if this is a factor in the response (or lack thereof) to selection, both direct and indirect. Could some of these robustness axes be decoupled and others not?Some general questions, not crucial to the results but perhaps good avenues for further investigation:- Could the coupling between axes of robustness be reduced in higher dimensional networks? Are the correlations weaker in the n=6 simulations than in the n=2 simulations?- Related to the previous point: would using larger networks allow for the inclusion of measures of network architecture? For example sparsity, modularity (...), and their relation to the correlations between robustness measurements.- Is the constitutive expression parameter relevant for the dynamics? How does setting different constitutive expression values alter the results? Could (should?) it be altered by mutations?- Could the difference in the evolvability in the different robustness measurements be due to the choice of mutation? i.e., Could a different, larger-mutation scheme, alter the evolvability?
Reviewed by Charles Mullon
, 03 Feb 2021
In gene network robustness as a multivariate character, the author uses computer simulations to investigate the evolution of various measures of robustness of gene expression. A gene regulatory network (based on the “Wagner” model) is evolved where the fitness of an individual depends on the capacity of the regulatory network to maintain a stable level of expression in the face of mutational or environmental perturbations. It is shown that selection for one type of robustness (e.g. against germline mutations) does not necessarily lead to a correlated response in another type of robustness (e.g. against late in life environmental perturbations). In fact, selection on different types of robustness can interfere with one another, slowing down or even preventing adaptation. Nevertheless, certain pairs of robustness types can be selected in different directions. This supports the notion that certain types of robustness are more evolvable than others (i.e. more independent from other traits than others).
This is an interesting manuscript, that is well written and easy to follow. The methods are particularly clear. In my opinion however there are a few points that would need to be addressed before recommendation.
I think that the concepts of pleiotropy and genetic constraints could be further investigated with a multivariate quantitative genetics framework (see the seminal paper by Lande in Evolution 1979 33(1)). In particular, estimating the G matrix of genetic variance-covariance for the different types of robustness would characterize genetic constraints in a more traditional and quantitative manner than it currently is.
Pleiotropy could also be quantified by computing the mutation matrix (e.g. Jones et al. Evolution 2007 61(4)). Doing so would also allow to connect the notions of robustness and evolvability more clearly. From reading the title and introduction, these quantitative genetics analyses are in fact the types of analyses that I expected to see.
I thought the description of results were terse and rather vague at times (e.g. p. 9-10, see sentences with “possible outcome ...”, “most cases ...”). Particularly disappointing is how little discussion there is about why different types of robustness are positively or negatively associated with one another. I understand this would be speculative without any further analyses, but it would nevertheless offer readers a message that goes beyond “it depends”. Perhaps the quantitative genetics approach suggested above allows for a more thorough description and interpretation of the associations between different types of robustness.
Minor comments / Typos:
Legend of figure 3: “robusnetss” -> robustness
Legend of figure 4: “univariare” -> univariate. In fact I did not understand what the thick horizontal lines corresponded to exactly.
Appendix 4 : “produced” -> produce
Appendix 5: There is something wrong here. I am guessing the rows and columns have been inverted in the legend. The column for stability also seems to be missing. Please check the figure legend thoroughly.